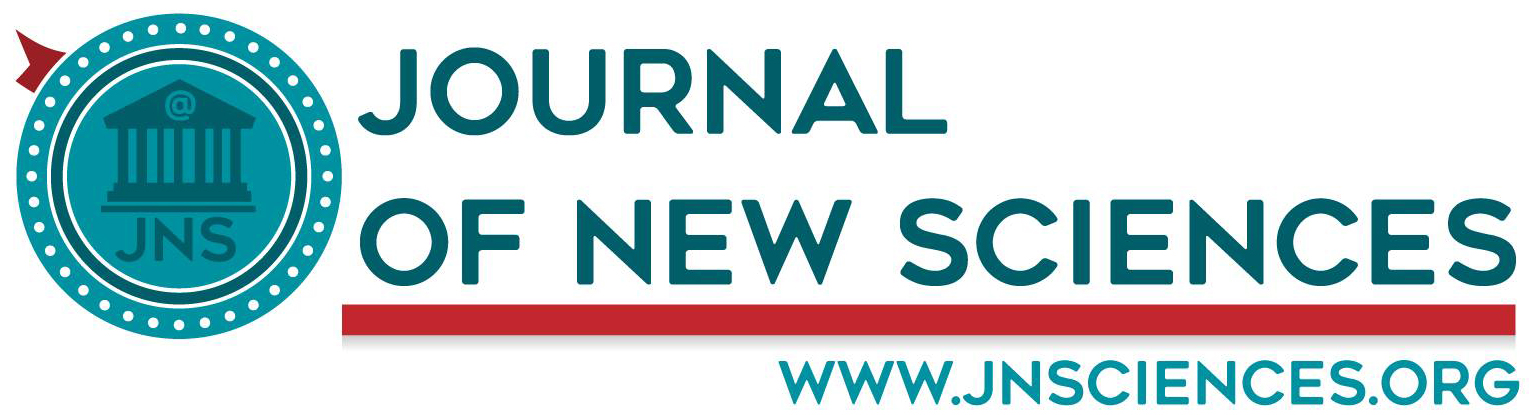
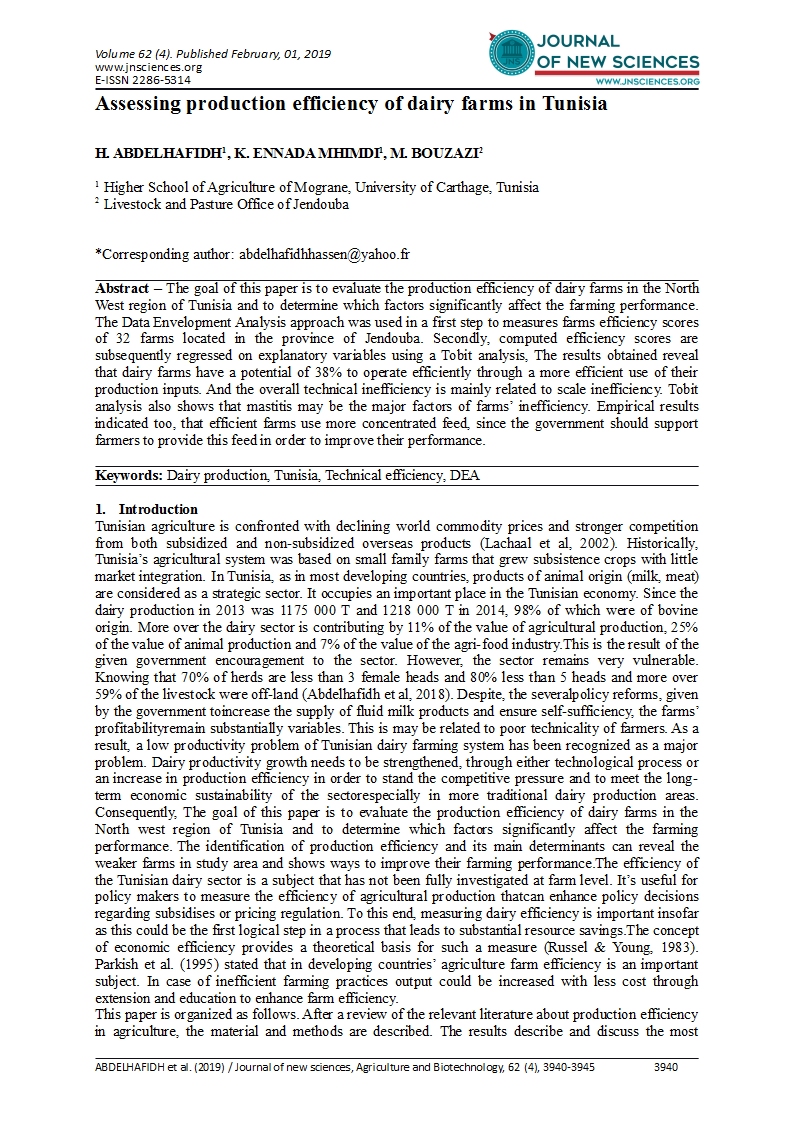
- Category: Volume 62
- Hits: 4713
Assessing production efficiency of dairy farms in Tunisia
H. ABDELHAFIDH1
K. ENNADA MHIMDI1
M. BOUZAZI2
1 Higher School of Agriculture of Mograne, University of Carthage, Tunisia
2 Livestock and Pasture Office of Jendouba
Abstract – The goal of this paper is to evaluate the production efficiency of dairy farms in the North West region of Tunisia and to determine which factors significantly affect the farming performance. The Data Envelopment Analysis approach was used in a first step to measures farms efficiency scores of 32 farms located in the province of Jendouba. Secondly, computed efficiency scores are subsequently regressed on explanatory variables using a Tobit analysis, The results obtained reveal that dairy farms have a potential of 38% to operate efficiently through a more efficient use of their production inputs. And the overall technical inefficiency is mainly related to scale inefficiency. Tobit analysis also shows that mastitis may be the major factors of farms’ inefficiency. Empirical results indicated too, that efficient farms use more concentrated feed, since the government should support farmers to provide this feed in order to improve their performance.
Keywords: Dairy production, Tunisia, Technical efficiency, DEA
1. Introduction
Tunisian agriculture is confronted with declining world commodity prices and stronger competition from both subsidized and non-subsidized overseas products (Lachaal et al, 2002). Historically, Tunisia’s agricultural system was based on small family farms that grew subsistence crops with little market integration. In Tunisia, as in most developing countries, products of animal origin (milk, meat) are considered as a strategic sector. It occupies an important place in the Tunisian economy. Since the dairy production in 2013 was 1175 000 T and 1218 000 T in 2014, 98% of which were of bovine origin. More over the dairy sector is contributing by 11% of the value of agricultural production, 25% of the value of animal production and 7% of the value of the agri-food industry.This is the result of the given government encouragement to the sector. However, the sector remains very vulnerable. Knowing that 70% of herds are less than 3 female heads and 80% less than 5 heads and more over 59% of the livestock were off-land (Abdelhafidh et al, 2018). Despite, the severalpolicy reforms, given by the government toincrease the supply of fluid milk products and ensure self-sufficiency, the farms’ profitabilityremain substantially variables. This is may be related to poor technicality of farmers. As a result, a low productivity problem of Tunisian dairy farming system has been recognized as a major problem. Dairy productivity growth needs to be strengthened, through either technological process or an increase in production efficiency in order to stand the competitive pressure and to meet the long-term economic sustainability of the sectorespecially in more traditional dairy production areas. Consequently, The goal of this paper is to evaluate the production efficiency of dairy farms in the North west region of Tunisia and to determine which factors significantly affect the farming performance. The identification of production efficiency and its main determinants can reveal the weaker farms in study area and shows ways to improve their farming performance.The efficiency of the Tunisian dairy sector is a subject that has not been fully investigated at farm level. It’s useful for policy makers to measure the efficiency of agricultural production thatcan enhance policy decisions regarding subsidises or pricing regulation. To this end, measuring dairy efficiency is important insofar as this could be the first logical step in a process that leads to substantial resource savings.The concept of economic efficiency provides a theoretical basis for such a measure (Russel & Young, 1983). Parkish et al. (1995) stated that in developing countries’ agriculture farm efficiency is an important subject. In case of inefficient farming practices output could be increased with less cost through extension and education to enhance farm efficiency.
This paper is organized as follows. After a review of the relevant literature about production efficiency in agriculture, the material and methods are described. The results describe and discuss the most important findings about the determinants of production efficiency of farms among North West region of Tunisia. The conclusions indicate the purpose and the main findings.
2. Materials and Methods
For the empirical procedures of this study, data envelopment analysis will be the method for calculating efficiency measures and benchmarking.
2.1. Efficiency measures and benchmarks: data envelopment analysis
The technique of data envelopment analysis (DEA) is a non-parametric approach, involving mathematical programming in its estimation, which was developed by the authors Charnes, Cooper and Rhodes (1978) for the relative efficiency analysis of producing units, known in the literature as DMUs (decision making unit). By producing unit is meant any system that transforms inputs into products. DEA aims at finding the best production unit, i.e. the one that combines resources more efficiently, so that it reaches the optimal production level (Pareto-Optimum). A production unit is efficient when there is no other unit maintaining the same level of output with lower level of inputs, or when there is no other unit achieving a higher level of output with the same level of inputs. Units with the highest efficiency are located on the efficient frontier (at the boundary of efficiency). The purpose of the DEA method is to construct a nonparametric envelopment frontier over the data points such that all observed points lie on or below the production frontier. The technical efficiency (TE) estimates vary between 0 and1.
The DEA models for estimating technical efficiency were based upon the assumptions of constant returns to scale (CRS) and variable return to scale (VRS) (Charnes et al., 1978; Banker et al., 1984). Overall technical efficiency measure (TECRS) was decomposed into pure technical efficiency (TEVRS) and scale efficiency (SE) for determining the source of inefficiency. The issue of returns to scale concerns what happens to units’ outputs when they change the amount of inputs they are using to produce their outputs. Under the assumption of variable returns to scale, a unit found to be inefficient has its efficiency measured relative to other units in the dataset of a similar scale size only.The initial assumption of the approach is that the measure of efficiency requires a common set of weights that will be applied to all DMUs. In order to select the optimal weights, a mathematical programming problem is specified for the i-th DMU.Under the non-parametric approach (DEA), to estimate the production frontier, we consider the “input oriented” model, according to Coelli (1996) : n farms (i=1,……n), each producing M outputs ymn (m=1,…M) by using K different inputs xkn(k=1,…..K), each farm becoming the reference unit. For the ith firm, we have vectors xi (K 1) and yi (M
1). For the entire data set, therefore, we have a K
N input matrix X and M
N output matrix Y. The technical efficiency (TE) measure is obtained by solving The CCR model which was initially proposed by Charnes et al., (1978). The CCR model is indicated in Eq. (1):
St
(1)
Where θi is a variable representing the efficiency of the Reference Farm i and hence the percentage of reduction to which each input must be subjected to reach theproduction frontier. λ is a vector of (k*1) elements representing the influence of each farm in determining the efficiency of the ith farm.
Thus, the linear programming problem with constant returns can be modified to meet the assumption of variable returns by adding the constraint of convexity, N1’λ=1, where N1 is a vector (n x 1) of unit numbers.
For each inefficient unit, DEA models provide their respective benchmarks, determined by the projection of these units at the efficiency frontier. This projection is done according to the orientation of the model, being orientation to inputs when it is desired to minimize the resources, keeping the values of the products constant, or orientation to products when it is desired to maximize the products without reducing the inputs (Abdelhafidh et al, 2017).
Solution to (1) provides information about the production efficiency of each individual farm of the sample and does not imply irrational behaviour of non-efficient farmers (Lachaal et al, 2002). The failure of farmers to produce potential output could be the result of factors linked to the farmer’s technicality or not. From a policy point of view, it is interesting to investigate the sources of inefficiency and to identify farm attributes potentially related to it. In some studies, production efficiency has been linked with a numerous of socioeconomic variables. However, more empirical research is still needed to highlight the relationship between efficiency and these attributes. Since, in this analysis, several variables are identified as potential determinants of technical efficiency.
These variable reflect the time present, the feed quality, the farmer age (Age), the education levelof farmer (NINS), the green fodder feed per dairy cow(GF), fodder area devoted per dairy cow (FAC) and the fight against mastitis (FM). To this end, the efficiency scores obtained are regressed on these farm attributes using the linear Tobit model in (2).
(2)
Where:
ET: is the efficiency of dairy farmers.
ε: is the error term.
2.2. Data collection
To implement the computation of technical efficiency scores in (1), data of 32 dairy farms, selected from 3 delegations in the province of Jendouba, Tunisia, collected on April 2018, were used in this study. The sample size was determined by means of simple random sampling method (Newbold, 1995). A structured questionnaire was used in collecting the data by personal interview. Basic descriptive statistics used in the analysis are presented at Table 1.
Regarding data required they are particularly related to milk production value as well as expenses of inputs used. Six broad categories of milk production inputs were considered. These were: herd population measured in heads (P), concentrate feed input (CC), fodder feed (F), veterinary charges (FV), material input (M) and labour input (L). The labor input included permanent and hired labor. The material input included equipments depreciation.
Table 1. Basic statistics of the variables used in the analysis of efficiency/farm/Year |
|||||
Variables |
Description |
Mean |
S.D |
MIN |
Max |
output |
Output Value(TND) |
41306 |
46687 |
4900 |
220000 |
inputs |
Cows presented : P( Nb of heads) |
11.4 |
8.9 |
2.0 |
47.0 |
Concentrates Feed Expenses :CC (TND) |
22563 |
20622 |
2300 |
110000 |
|
fodder feed Expenses: F (TND) |
12016 |
9872 |
4700 |
54000 |
|
Veterinary Charges :FV (TND) |
1001 |
263 |
420 |
1400 |
|
Material Input :M (TND) |
872 |
296 |
200 |
1300 |
|
Labour Expenses : L (TND). |
5281 |
1778 |
3600 |
8500 |
|
Specifics factors |
Age (years) |
49 |
13 |
22 |
68 |
Education level: NINS (0 if the education level was lower or equal to primary , 1 : if secondary, 2: if University level) |
0 : 41% |
|
|
||
1: 38% |
|||||
2: 7% |
|||||
Quantity of green fodder given to the herd: GF (kg) |
228209 |
241812 |
0 |
1216125 |
|
Fodder area devoted per dairy cow :FAC (ha) |
0.49 |
0.52 |
0 |
3 |
|
Fight against mastitis: FM (1: if yes,0: if no). |
0,59 |
0,50 |
0 |
1 |
3. Results and Discussion
Using the DEA methodology outlined above, non-parametric analysis of relative technical efficiency is performed for dairy production in the farms of the sample. Average overall technical efficiency is about 62%. This means that the farms can increase their milk production by as much as 38% using the same production inputs more efficiently. Pure technical and scale efficiency measures indicated that overall inefficiency was mainly due to scale inefficiency. Technical efficiency was possible to have been increased from 0.62 to 0.95 if scale inefficiency did not exist (Table 2).
Table 2. Technical, scale, efficiency measures |
|||
Efficiency measures |
Mean |
Standard deviation |
Efficient farms(%) |
Overall technical efficiency |
0,62 |
0,19 |
13% |
Pure technical efficiency |
0,95 |
0,09 |
69% |
Scale efficiency |
0,65 |
0,18 |
13% |
Results schow too, that 13% of farms are operating CRS scale against 87% operating in VRS and all of them are in increasing return to scale. Scale efficient farms (Constant return to scale-CRS) had higher gross production value in comparison to farms with increasing return to scale (IRS) (Table 3).
Table 3. Gross production values for different scales
|
||
Return to Scale |
% of farms |
Gross production value (TND/Cow) |
Constant return to scale |
13% |
5050 |
Increasing return to scale |
87% |
3058 |
Results presented in table 4 show that average herds owned by efficient farms is three times more than those owned by the inefficient ones. Efficient farms use 16% of concentrates feed/cow more than those inefficient.
Table 4. Comparison between efficient and inefficient farms |
||
Inputs |
Economic efficiency |
|
Efficient Farms |
Inefficient Farms |
|
Cows presented |
27 |
9 |
Concentrates Feed Expenses (TND) |
59250 |
17321 |
fodder feed Expenses (TND) |
26550 |
9939 |
Veterinary Charges (TND) |
1120 |
984 |
Material Input (TND) |
950 |
861 |
Labour Expenses (TND). |
5725 |
5218 |
Comparison of current and optimum uses of major inputs showed that it was possible to maintain the current gross output value while decreasing the number of cow, concentrated feed (kg), Fodder Feed, veterinary expenses, materiel expenses and labor (Table 5).
It is recommended for inefficient farms to benchmark in an effort to achieve similar efficiency levels of efficient farms with minimum input levels or by improving Gross production values with the same amount of inputs. Inefficient dairy farms should be encouraged by convenient state policies in this regard.
Table 5. The comparison between current and optimum input levels per cow and possible changes |
||||||
|
P |
CC |
F |
FV |
M |
L |
Current |
11 |
22563 |
12016 |
1001 |
872 |
5281 |
optimum |
10 |
19378 |
10299 |
912 |
750 |
4825 |
changes |
14% |
14% |
14% |
9% |
14% |
9% |
To identify factors associated with technical inefficiencies, the T obit regression defined in equation
(2) is estimated using Stata package and results are presented in table 6.
Regarding the Tobit model results, the likelihood ratio test rejects a null hypothesis that all slope parameters are simultaneously null. This confirms that the Tobit model is statistically valid. Overall technical efficiency is positively affected by all explaining factors.
The Tobit model results also indicated that fighting Mastitis has the most marginal effect on technical efficiency with a coefficient of 0.208 and significant at the 1% level. This result indicated that when farmers give more attention to avoid mastitis with in his herd may enhance his efficiency by about 21%. Results also show that education level (NINS), has an important effect on technical efficiency. Since its coefficient is 0.088 and significant at 5% level implying that the change of the education level by one point enhances efficiency by 8.8%. This means that government has to improve farmers’ knowledge and information by execution of specifics formation sessions and narrow technical support, especially for non educated farmers. While age and Fodder area devoted per dairy cow tend to increase efficiency but are not significant at the 10% level. Despite it is significant at 1% level, the quantity of green fodder given to the herd has poor effect because its coefficient is slightly superior to zero.
Table 6. Tobit estimation results of factors affecting technical efficiency scores |
|||
variables |
Coefficients |
SD |
t-statistic |
Age (years) |
0.003 |
0.002 |
1.30 |
Education level: NINS |
0.088** |
0.035 |
2.47 |
Quantity of green fodder given to the herd: GF (kg) |
0.0001* |
0.0001 |
2.89 |
Fodder area devoted per dairy cow : FAC (ha) |
0.085 |
0.054 |
1.54 |
Fight against mastitis: FM (1: if yes, 0: if no). |
0.208* |
0.063 |
3.28 |
Constant |
0.137 |
0.145 |
0.94 |
LR chi2 |
26.58 |
||
Prob> chi2 |
0.0001 |
||
Log-likelihood |
9.208 |
||
*Significant at 1% level; ** significant at 5% level |
4. Conclusion
In this paper, DEA approach was used to measure the technical efficiency scores for a sample of Tunisian dairy farms located in the north west region in first step. The second step allows to investigate the sources of technical efficiency within the sample and to identify potential farm attributes related to it. Empirical results show that significant inefficiencies in dairy production are found within the sample of farms at hand. In particular, 87% of the farms are found to produce below of their potential due to technical inefficiency. Since, overall efficiency measure suggests that farms in Tunisia could increase milk production by as much as 38% through a more efficient use of their production inputs. This much explains the shortage of milk supply in the country. The relationship between efficiency and five explicatory factors is assessed by resorting to Tobit analysis. Findings suggested that education and fight against mastitis were the most influencing factors to enhance farms’ productivity. Empirical results also indicated that efficient farms use more concentrated feed, since the government should support farmers to provide this feed in order to improve their efficiency.
5. References
Abdelhafidh H., Abdelfattah I., Arfa L., 2018. The role of the service cooperative in attenuating the transaction costs in dairy farms: a case study from Tunisia. Journal of new sciences, Sustainable Livestock Management, 6 (2), 115-123.
Abdelhafidh H., Ben Brahim M., Abed H., Mekki A. 2017. Analysis of the technical efficiency, pure and scale efficiency of rained cereal farms: Case of the upper semi-arid. Journal of Experimental Biology and Agricultural Sciences, August - 2017; Volume – 5.
Banker RD, Chames A, Cooper WW (1984) Some models for estimating technical and scale efficiencies in data envelopment analysis. Management Science 30. 1078-1092.
Charnes A., Cooper W.W., Rhodes E. (1978). Measuring the efficiency of decision making units. European Journal of Operational Research, 2(6): 429-444. DOI: 10.1016/0377- 2217(78)90138-8
Coelli TJ (1996) A guide to DEAP Version 2.0: a data envelopment analysis (computer) program. Center for Efficiency and Productivity Analysis (CEPA) Working Paper 96/ 08. Department of Econometrics. University of New England, Armidale, Australia.
Lassaad Lachaal, Najet Chahtour, Boubaker Thabet, (2002). Technical efficiency of dairy production in Tunisia: a data envelopment analysis. NEW MEDIT N. 3 (2002)
Newbold, P. (1995). Statistics for Business and Economics. Prentice-Hall, New Jersey.
Parkish, A., Ali, F., & Shah, M. K. (1995). Measurement of economic efficiency in Pakistani agriculture. Amer. J. Agr. Econ., 77, 675 - 85. http:dx.doi.org/10.2307/1243234.
Russell, N. P., & Young, T. (1983). Frontier production functions and the Measurement of technical efficiency. J. Agr. Econ., 34, 139-50.