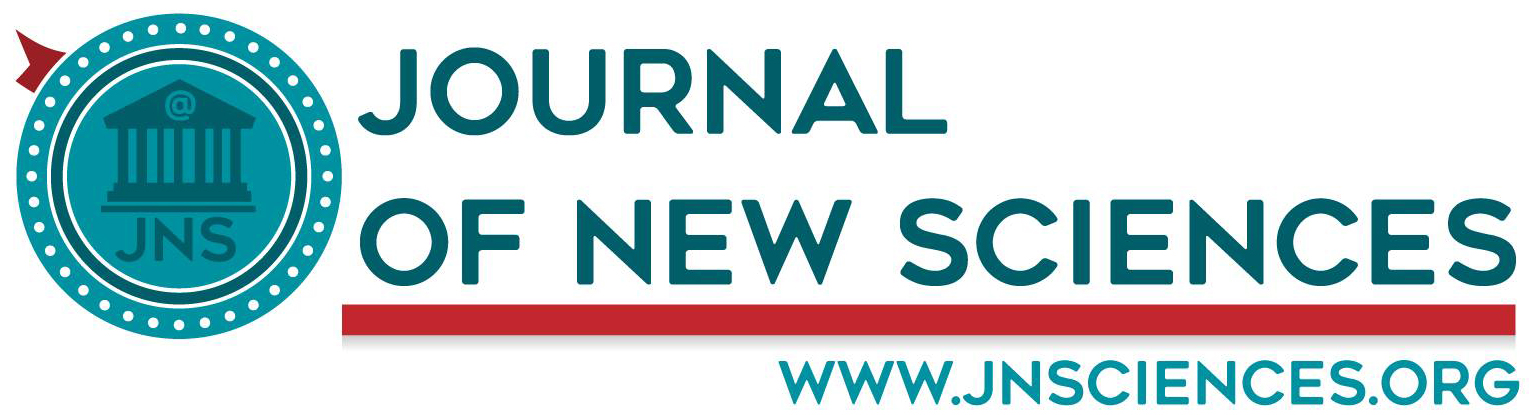
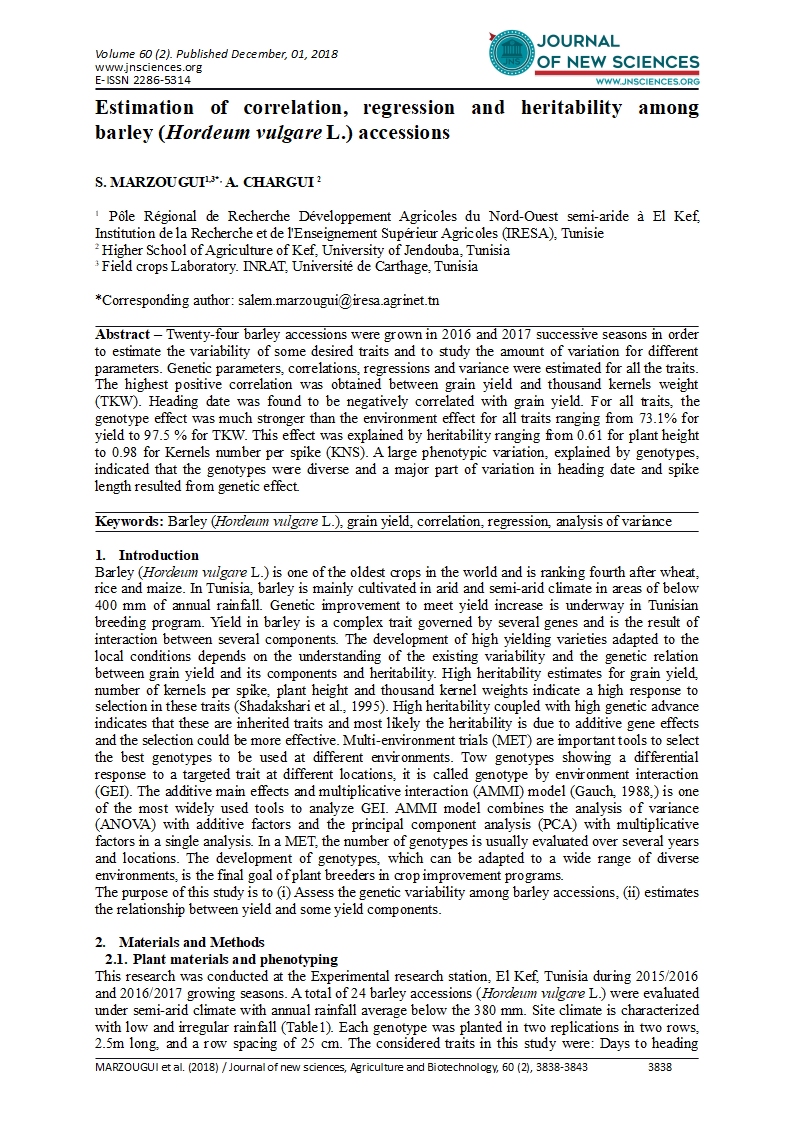
- Category: Volume 60
- Hits: 4642
Estimation of correlation, regression and heritability among barley (Hordeum vulgare L.) accessions
S. MARZOUGUI1,3
A. CHARGUI 2
1 Pôle Régional de Recherche Développement Agricoles du Nord-Ouest semi-aride à El Kef, Institution de la Recherche et de l'Enseignement Supérieur Agricoles (IRESA), Tunisie
2 Higher School of Agriculture of Kef, University of Jendouba, Tunisia
3 Field crops Laboratory. INRAT, Université de Carthage, Tunisia
Abstract – Twenty-four barley accessions were grown in 2016 and 2017 successive seasons in order to estimate the variability of some desired traits and to study the amount of variation for different parameters. Genetic parameters, correlations, regressions and variance were estimated for all the traits. The highest positive correlation was obtained between grain yield and thousand kernels weight (TKW). Heading date was found to be negatively correlated with grain yield. For all traits, the genotype effect was much stronger than the environment effect for all traits ranging from 73.1% for yield to 97.5 % for TKW. This effect was explained by heritability ranging from 0.61 for plant height to 0.98 for Kernels number per spike (KNS). A large phenotypic variation, explained by genotypes, indicated that the genotypes were diverse and a major part of variation in heading date and spike length resulted from genetic effect.
Keywords: Barley (Hordeum vulgare L.), grain yield, correlation, regression, analysis of variance
1. Introduction
Barley (Hordeum vulgare L.) is one of the oldest crops in the world and is ranking fourth after wheat, rice and maize. In Tunisia, barley is mainly cultivated in arid and semi-arid climate in areas of below 400 mm of annual rainfall. Genetic improvement to meet yield increase is underway in Tunisian breeding program. Yield in barley is a complex trait governed by several genes and is the result of interaction between several components. The development of high yielding varieties adapted to the local conditions depends on the understanding of the existing variability and the genetic relation between grain yield and its components and heritability. High heritability estimates for grain yield, number of kernels per spike, plant height and thousand kernel weights indicate a high response to selection in these traits (Shadakshari et al., 1995). High heritability coupled with high genetic advance indicates that these are inherited traits and most likely the heritability is due to additive gene effects and the selection could be more effective. Multi-environment trials (MET) are important tools to select the best genotypes to be used at different environments. Tow genotypes showing a differential response to a targeted trait at different locations, it is called genotype by environment interaction (GEI). The additive main effects and multiplicative interaction (AMMI) model (Gauch, 1988,) is one of the most widely used tools to analyze GEI. AMMI model combines the analysis of variance (ANOVA) with additive factors and the principal component analysis (PCA) with multiplicative factors in a single analysis. In a MET, the number of genotypes is usually evaluated over several years and locations. The development of genotypes, which can be adapted to a wide range of diverse environments, is the final goal of plant breeders in crop improvement programs.
The purpose of this study is to (i) Assess the genetic variability among barley accessions, (ii) estimates the relationship between yield and some yield components.
2. Materials and Methods
2.1. Plant materials and phenotyping
This research was conducted at the Experimental research station, El Kef, Tunisia during 2015/2016 and 2016/2017 growing seasons. A total of 24 barley accessions (Hordeum vulgare L.) were evaluated under semi-arid climate with annual rainfall average below the 380 mm. Site climate is characterized with low and irregular rainfall (Table1). Each genotype was planted in two replications in two rows, 2.5m long, and a row spacing of 25 cm. The considered traits in this study were: Days to heading (DH), plant height (PH), Spike length (SL), Kernels number per spike (KNS), thousand kernels weight (TKW) and grain Yield (Yld).
Table 1. Description of the environment during two cropping seasons |
||||||||
Years |
|
Nov. |
Dec. |
Jan. |
Feb. |
Mar. |
Apr. |
May |
|
Average T. (°C) |
Vegetative stage |
Flowering stage |
Grain filling stage |
||||
2017/2018 |
14.0875 |
11 |
7.9 |
9.7 |
8.1 |
12.2 |
15.9 |
25 |
2016/2017 |
14.2625 |
13.4 |
10.5 |
6.3 |
9.4 |
11.9 |
15.7 |
20.8 |
|
Precipitations (mm) |
|
|
|
|
|
|
|
2015/2016 |
270.47 |
82.5 |
36.8 |
32.5 |
31.7 |
41.2 |
36.3 |
48.5 |
2016/2017 |
221.71 |
44.2 |
53.1 |
35 |
24.9 |
4.31 |
17.5 |
2.3 |
|
Days with precipitation (days) |
|
|
|
|
|
|
|
2015/2016 |
55 |
8 |
11 |
4 |
16 |
15 |
10 |
6 |
2016/2017 |
54 |
7 |
12 |
13 |
5 |
5 |
7 |
1 |
|
Humidity (%) |
|
|
|
|
|
|
|
2015/2016 |
63.8125 |
70.8 |
77.8 |
69.7 |
74.4 |
66 |
61.8 |
52.3 |
2016/2017 |
62.5375 |
68.9 |
81.8 |
76.7 |
73.4 |
63.5 |
53.1 |
42.6 |
2.2. Statistical Analysis
2.2.1. Descriptive statistics
For each trait, descriptive statistical measures were obtained based on the average data of the barley lines. Correlation matrix between all traits was performed using the R package: Performance Analytics
2.2.2. Heritability and regression
Genetic correlation among variables, heritability and the estimation of the genetic effects were estimated using Multi Environment Trial Analysis with R (META-R) (Alvarado et al., 2016). To analyze the relationships between grain yield and yield components accurately, the regression analysis was performed for all genotypes using STAD-R version 1.0 (Pacheco et al., 2016).
2.2.3. Analysis of variance
The additive main effects and multiplicative interaction (AMMI) model combines two standard methods: Analysis of variance (ANOVA) with principal components (PC) analysis (Zobel et al. 1988). Therefore, Gauch, (1992) express the model equation for the i-th genotype in the j-th environment (repetitions) as:
r
Where Yijr is the phenotypic trait; is the grand mean; gi is the main effect of the ith genotype (G); ej is the main effect of the jth environment (E). λk is the singular value for the Interaction Principal Component (IPC) axis k, αik and γjk are the genotype and environment IPC scores (i.e. the left and right singular vectors) for axis k, br (ej ) is the effect of the block r within the environment j, r is the number of blocks (In this study number of blocks was not considered, then the effect of blocks as removed), ρij is the residual containing all multiplicative terms not included in the model, n is the number of axes or principal components (PC) retained by the model, and εijr is the experimental error, assumed independent with identical distribution εij ∼ N (0, σ).
3. Results and Discussion
This study was conducted kef region characterized by low and irregular rainfall distribution during the cropping season especially at the grain filling stage which affect grain yield as mentioned in table 1. During flowering and grain filling stage, the total rainfall dropped from 47.5 mm within 13 days in 2016 to 19.8 mm in 8 days in 2017. Because of this irregular rainfall distribution, we have considered each year as a separate environment.
Basic statistical parameters in each year (mean values, standard deviation; minimum and maximum values) for the 24 barley lines are summarized in table 2. Some traits showed a decrease in their mean value between the two cropping seasons. Means of grain yield decreased from 247 and 216 g/m2. Plant height dropped from 79 cm to 72 cm. However, there was a delay in the flowering time from 127 days in 2016 to 131 days in 2017. Other traits showed a stable mean value across the two years like Spike length (SL) and thousand kernels per spike (TKW).
Table 2. Descriptive statistics of the studied traits |
|||||
Trait |
Year |
Mini. |
Max. |
Mean |
SDV |
DH |
2016 |
114 |
148 |
127.7292 |
10.0905 |
DH |
2017 |
118 |
152 |
131.75 |
9.461 |
PH |
2016 |
63 |
95 |
78.9833 |
9.0507 |
PH |
2017 |
60 |
81 |
72.25 |
5.6286 |
SL |
2016 |
4.9 |
10.1 |
7.6262 |
1.3937 |
SL |
2017 |
5.2 |
11.2 |
7.8563 |
1.6776 |
KNS |
2016 |
22 |
98 |
58.7708 |
19.7921 |
KNS |
2017 |
22 |
100 |
57.0042 |
19.9103 |
Yld |
2016 |
110 |
569 |
246.9792 |
127.7743 |
Yld |
2017 |
78 |
374 |
215.875 |
82.0157 |
TKW |
2016 |
22.8 |
42.3 |
31.8083 |
5.131 |
TKW |
2017 |
21.3 |
42.3 |
31.5521 |
5.1847 |
3.1. Correlation and regression analysis
Genetic and phenotypic correlations of the studied traits across the two cropping seasons are presented in Table 3. The combined data over two years show that thousand kernels weight (TKW) has a significant positive genetic correlation with grain yield (r= 0.44), but it is negatively correlated with days to heading(r=-0.80) and kernels number per spike (KNS) (r= -0.39). These data measure the strength of the relationship between the genetic values of both traits with yield and the existence of pleiotropic effects. Phenotypic correlation coincides with genetic correlation data for almost all traits except the significant correlation between plant height and yield (r= 0.40).The results of the correlation analysis suggest that early heading plant is associated with increase in plant height, thousand kernels weight and grain yield.
Table 3. Genetic and phenotypic correlations across two cropping seasons.
|
|||||
(a) |
|||||
Traits |
DH |
PH |
SL |
KNS |
Yld |
PH |
-0.44* |
|
|
|
|
SL |
0.35* |
-0.44* |
|
|
|
KNS |
0.32 |
-0.11 |
0.11 |
|
|
Yld |
-0.80* |
0.29 |
-0.20 |
-0.39* |
|
TKW |
-0.36* |
-0.01 |
-0.27 |
-0.30 |
0.44* |
(b) |
|||||
Traits |
DH |
PH |
SL |
KNS |
Yld |
PH |
-0.40* |
|
|
|
|
SL |
0.33 |
-0.27
|
|
|
|
KNS |
0.30 |
-0.08 |
0.19 |
|
|
Yld |
-0.69* |
0.40* |
-0.15 |
-0.19* |
|
TKW |
-0.35* |
0.05 |
-0.23 |
-0.29 |
0.42* |
* Correlation is significant at the 0.05 level |
Simple regression coefficients of yield on the different traits were analyzed and are presented in table 4 and figure 1.
|
Figure 1. Regression analysis between grain yield and yield related traits. |
Regressions were positive for plant height and thousand kernels weight (TKW) indicating that the increase in these traits will increase grain yield. However, negative regressions were found for heading date and spike length. The, heading date and the plant height, showed the highest adjusted R2, 0.34 and 0.16 respectively, indicating their important role in barley grain yield in the two cropping seasons. The regression equation shows that the coefficient for days to heading is -6.4 days. This coefficient indicates that for every additional day in heading we can expect yield to decrease by 6.4 g/m2. The variation of the grain yield could be also explained by the variation of plant height. For each increase in the plant height by 1cm we can expect yield to increase by 5.4g/m2.
Table 4. Simple linear regression of different traits on yield |
|||||
Traits |
Regression values (b value) |
R2 adjusted |
SE of coeff. |
t value |
P-value |
Days to heading (DH) |
-6.4* |
0.34 |
0.9 |
-7.1 |
0 |
Plant height (PH) |
5.4* |
0.16 |
1.2 |
4.38 |
0 |
Spike length (SL) |
-2.2 |
-0.01 |
7.2 |
-0.3 |
0.7 |
Kernels number per spike (KNS) |
-0.89 |
0.01 |
0.5 |
-1.6 |
0.11 |
Thousand kernels weight (TKW) |
7.6* |
0.12 |
2 |
3.8 |
0 |
* Significant at the 0.05 level |
Multiple linear regressions for all traits were highly significant except for Kernels number per spike (KNS) (Table 5). This analysis was carried out to build a model to explain the total variation of grain yield (R2=0.43). The equation for yield based on the studied traits is as following:
Y=434.5-2.25x1+3x2+14.5x3-0.13x4+4.5x5
Table 5. Multiple linear regression to analyze the effect of studied traits on yield |
|||||
Traits |
Regression values (b value) |
R2 adjusted |
SE of coeff. |
t value |
P-value |
Constant |
434.5 |
0.43 |
200 |
2.1 |
0.03 |
Days to heading (DH) |
-2.25 |
1 |
-5.1 |
0 |
|
Plant height (PH) |
3 |
1 |
2.7 |
0.08 |
|
Spike length (SL) |
14.5 |
5.7 |
2.5 |
0.015 |
|
Kernels number per spike (KNS) |
-0.13 |
0.45 |
-0.3 |
0.7 |
|
Thousand kernels weight (TKW) |
4.5 |
1.75 |
2.5 |
0.01 |
3.2. Heritability and analysis of variance
The analysis of variance for grain yield related traits of each genotype in each year based on GxE interaction effects is presented in (Table6). The genotypes, environment (seasons) and the interaction effects GxE interactions were highly significant (P ≤ 0.01) of the total variance. The genotype effect was much stronger than the Environment effect for all traits ranging from 73.13%% for yield to 97.5 % for TKW. This effect was explained by heritability ranging from 0.61 for plant height to 0.98 for KNS. A large phenotypic variation, explained by genotypes, indicated that the genotypes were diverse and a major part of variation in heading date and spike length resulted from genetic effect.
Table 6. Heritability, genotype and environment variance across two cropping seasons |
|||||||
Traits |
Genotype variance |
Env. variance |
Gen*Env variance |
Genotype significance |
Gen*Env significance |
Heritability |
|
Days to heading (DH) |
87.64 |
4.17 |
8.17 |
0.00 |
0.0 |
0.91 |
|
Plant height (PH) |
59 |
17.54 |
23.17 |
0.03 |
0.0 |
0.61 |
|
Spike length (SL) |
94.1 |
0.57 |
4.6 |
0.00 |
0.0 |
0.95 |
|
Kernels number per spike (KNS) |
97.5 |
0.2 |
2.2 |
0.00 |
0.0 |
0.98 |
|
Thousand kernels weight (TKW) |
88.45 |
0.06 |
11.47 |
0.00 |
0.0 |
0.87 |
|
Grain yield (Yld) |
73.13 |
2.1 |
24.75 |
0.01 |
0.0 |
0.66 |
Days to heading, plant height, spike length and kernels number per spike are the most important agronomic traits that can be used to select and develop high-yielding genotypes adapted to drought conditions. In this research, the correlation between yield and the related traits showed a positive correlation between plant height and yield (r=0.40). According to Drikvand et al. (2011) and Niazi-Fard et al. (2012), a significant and positive correlation between grain yield and plant height was also observed. The correlation of number of grains per spike with 1000-grain weight was negative suggesting that the increase of spike length increase the number of grains per spike and decreased the 1000 grain weight. A negative correlation between heading date and yield showed that early heading lines have a high yield. In such conditions, grain filling of late heading date cultivars often occurs in unfavorable environments characterized by high temperatures and water deficit in semi-arid climate. In this study, high estimates of heritability were shown for all traits except for grain yield and plant height. Ehdaie and Waines (1989) suggested that heritability estimates of grain yield are usually lower than other characters, because the environmental effects constitute a major portion of the total phenotype variation in this character.
4. Conclusion
From this study, a significant genotypic variation is present among barley lines to be subjected to selection for grain yield. The data obtained from this study could be useful for future breeding barley programs.
Therefore, the studied traits could be used as selection criteria to increase grain yield in barley.
Acknowledgments
This research was supported by a grant from Korea-Africa Food and Agricultural Cooperation Initiative (KAFACI).
5. References
Ángela Pacheco, Francisco Rodríguez, Gregorio Alvarado, Mateo Vargas, Marco López, José Crossa, Juan Burgueño(2017) "STAD-R Descriptive statistics for experimental designs", hdl:11529/10853, CIMMYT Research Data & Software Repository Network, V3
Drikvand R, Samiei K and Hossinpor T (2011) Path Coefficient negative direct effect (-0.634) on grain yield. Our results are in Analysis in Hull-less Barley under Rainfed Condition. Australian Journal of Basic and Applied Sciences, 5, 12, 277-279
EHDAIE, B. and J.G. WAINES (1989) Genetic variation, heritability and path-analysis in landraces of bread wheat from southwestern Iran. Euphytica 41:183-190
Francisco Rodríguez, Gregorio Alvarado, Ángela Pacheco, José Crossa, Juan Burgueño (2017) "Spatial META-R. Spatial Multi-Environment Trial Analysis with R for Windows. Version 2.0", hdl: 11529/10850, CIMMYT Research Data & Software Repository Network, V3
Gauch H G (1988) Model selection and validation for yield trials with interaction. Biometrics 44:705–715
Niazi-Fard A, Nouri F, Nouri A, Yoosefi B, Moradi A, Zareei A (2012) Investigation of the relationship between grain yield and yield components under normal and terminal drought stress
Shadakshari YG, Virupakshappa K, Shivashankar G (1995) Genetic variability studies in the germplasm collections of sesamum (Sesamum indicum L). Mysore Journal of Agricultural Science 29:133-137.
Zobel, RW, Wright, MJ and Gauch, HG (1988) Statistical analysis of a yield trial. Agronomy Journal 80:388-393