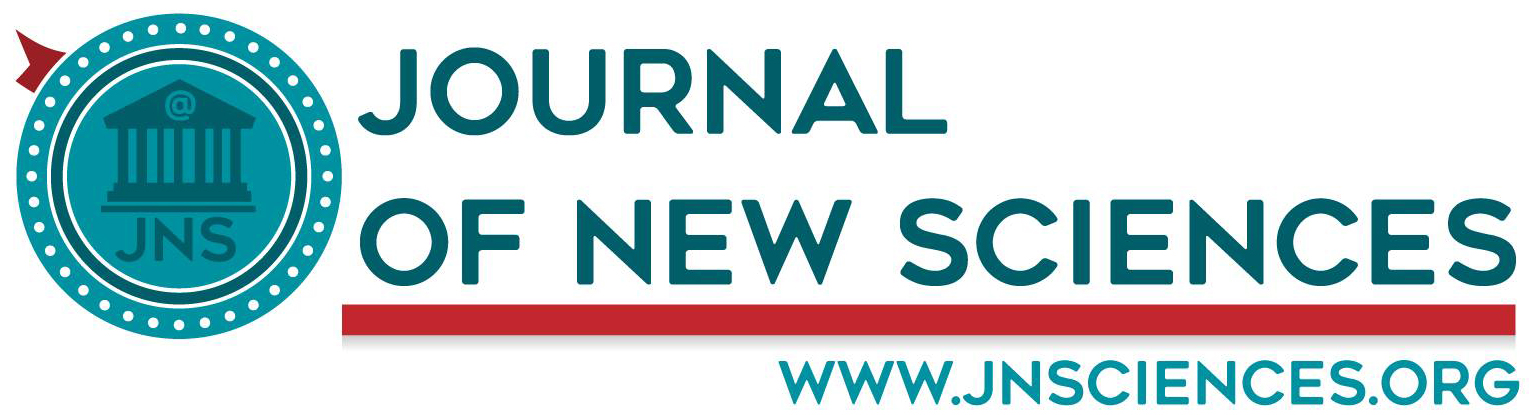
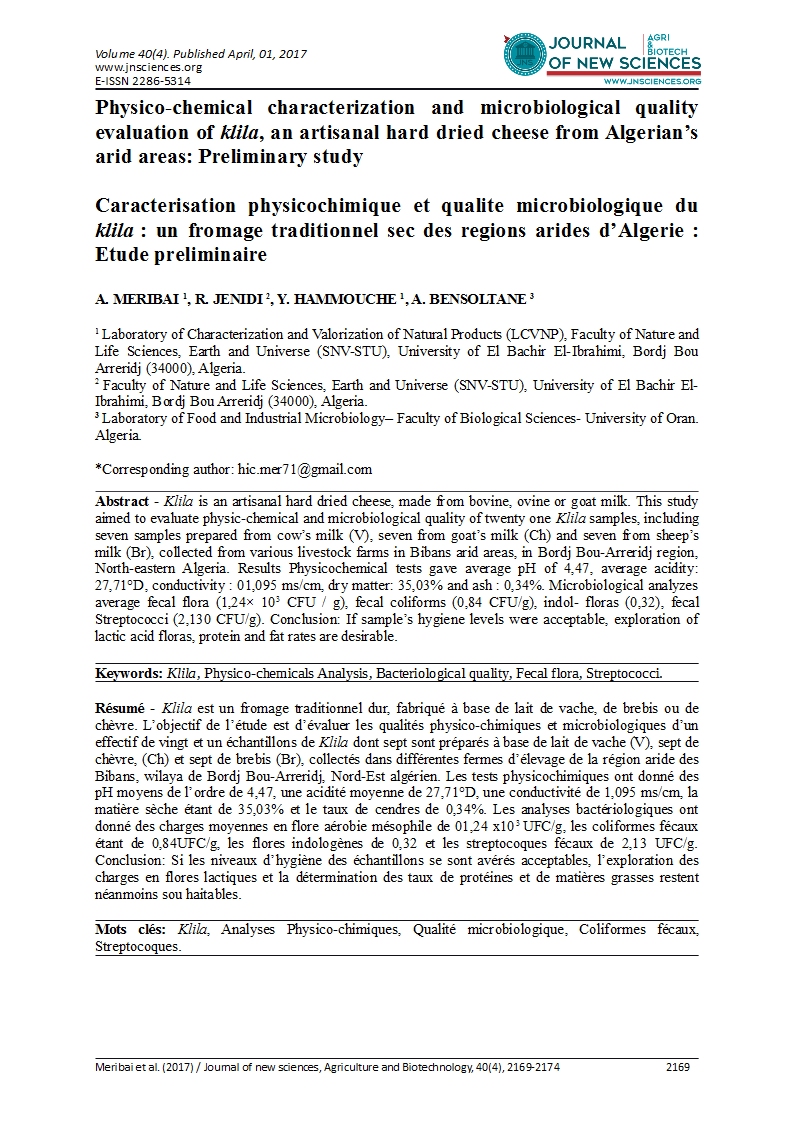
- Category: Volume 41
- Hits: 12298
Effect of water users associations’ financial performances on small farms’ productivity
H. ABDELHAFIDH1
M. S. BACHTA2
1Ecole supérieure d’Agriculture de Mograne, Université de Carthage, Carthage, Tunisia
2Institut National Agronomique de Tunis, Université de Carthage, Carthage, Tunisia
Abstract - Irrigation water management in Tunisia was devoted to water users associations (WUAs) under specific institutional arrangements based on users’ participation with the purpose to promote, financial viability and water savings. However, water fees were in many cases low and did not allow covering fixed costs of water production, which led to budget deficits of many of these WUAs. This vulnerable financial situation generates technical difficulty and poor delivery services to farmers affecting their productivity. This paper aims to evaluate the scope and the impact of WUAs’ financial deficits on their respective farms’ production efficiencies. A non-parametric Data Envelopment Analysis (DEA) approach was applied to measure farms’ efficiency scores. Tobit regression was then used to regress the farmers’ efficiency scores to the budget deficit of their corresponding WUA and the water turn time. Results indicate that average technical, allocative and economic efficiencies under constant return assumption to scale are respectively about: 68%, 71% and 48%. This is showing a considerable potential for enhancing farms’ profitability by reducing costs through improved farms’ efficiency. By operating on full economic efficiency levels the farmers would be able to reduce in average, their cost by 52%. Results also show that budget deficit of corresponding WUAs and water turn time have both negatives and significant effects on farms’ efficiencies. This indicates that improvement of local organizational performances leads to better farms’ performances.
Keywords: Tunisia, economic efficiency, DEA method, Tobit, Water pricing, WUA, Irrigation
- Introduction
Population growth and rising living standards have led to a rapid increase in the demand for water. As the annually renewable fresh water available in any particular location is on average constant and water conveyance is an expensive operation, water has become scarce in many parts of the world. Adding the prevalence of deteriorating water quality and the increased awareness for water-related environmental and social problems explains why water resource management has become a critical policy issue in many countries. Worldwide, irrigation consumes the most of the available renewable fresh water resources. Irrigated areas are expected to further expand and the irrigation sector, which already consumes a large share of global water, will thus continue to increase its demand in the foreseeable future. A common ground in past policy schemes was the development of appropriate irrigation infrastructure to enhance water supply and delivery. However, these expansionary policies have led to a massive use of irrigation water at a heavily subsidized cost, and to an increasing scarcity of the resource. Water shortage has become a major social and economic concern for policy makers and for users who competing for these resources. In Tunisia, water reserves are estimated at 4.8 billion m3/year, of which 2.7 billion m3 come from surface water flows (mostly located in the north), 0.7 billion m3 from shallow groundwater aquifers (in the centre, the plains and the coastal area), and approximately 1.3 billion m3 from the deep groundwater table (mainly in the south) (Al Atiri, 2005). Water resources are unevenly distributed across the country, with around 60% located in the north, 18% in the centre and 22% in the south. Taking into account the limited water resources and the frequent disparity between supply and demand during dry seasons, Tunisia has engaged, over the last three decades, in dynamic of water mobilization. Several investments have been granted, reaching 9% of total investments in the government’s Development Plan XI (2007-2011), 19% in water programs (Mahdhi and al, 2014). Irrigation consumes about 80% of the available water resources and contributes by 35% of the output value derived from the agricultural sector, 22% of exports, and 26% of agricultural employment. The efficiency of the irrigation networks is relatively weak, estimated at approximately 50% (Bachta and Ghersi, 2004). Therefore, during the recent decades concerns regarding the efficient use of water resources in the country have increased. These concerns have been addressed particularly to the transfer of public water management systems to water user associations.( WUAs).
WUAs have been created by government financing but they are responsible to ensure the collection of the water fees as well as service-related fees (infrastructure maintenance, etc.) after more than two decades of their creation, WUAs in Tunisia are still facing severe financial difficulties, related to unpaid water bills (the rate of collection of water bills, 1n 2012 does not exceed 40 % of the total volumes supplied to farmers) (Abdelhafidh and Bachta, 2016). This situation has created a negative impact on the ability to maintain distribution channels and water supply of the members (Gana and Fouillen 2013). In fact, the WUAs set the price at the marginal-cost exclusively (operational and maintenance cost or cost of water delivery). This method of pricing does not allow covering the costs of investments that are quite high.
In fact, the WUAs have to determine the water price at the beginning of the agricultural season and to decide whether the payment is on the basis of water volumes to be produced or distributed. Furthermore, they establish the water price based on the operation and maintenance cost. Financially, WUAs perform the following tasks: operation and maintenance of canals, repairing of various infrastructures, functioning of the association and investments. The water charge established by the WUAs comprises water-purchase charges, energy fees, labour force charges and maintenance and management fees.
The WUAs are considered to be natural monopolies and pricing at the operational cost which is considered by economists as pricing that enables optimal resource allocation (the one that maximizes social welfare) does not allow full cost recovery and lead to a chronic budgetary deficit of the WUAs. Lower cost recovery and poor maintenance of the irrigation network caused infrastructure deterioration, low water distribution efficiency and irrigation performances (Easter and Liu 2005). Pricing irrigation water based on the operational cost that’s very small compared to the actual value of the resource and to the full cost of supply (fixed and O&M cost). Indeed, this method of pricing does not reflect the growing water scarcity and does not lead to changes in farm’s behaviour towards a more efficient use of irrigation water. Already 30 years have passed after the transfer of water management to WUAs. These WUAs are still subsidized and they face many challenges related to technical, financial and social aspects (Bachta and Zaibet, 2007). These concerns vary from a WUA to another and affect by consequence the farms performance.
The purpose of this paper is to evaluate the scope and the impact of WUAs’ financial deficits on their respective farms’ production efficiencies. We assume that the improvement of the WUAs’ financial situation will positively affect the farms’ performance.
In our case the relative efficiency is measured via the nonparametric “Data Envelopment Analysis” (DEA) technique. Firstly the DEA models are constructed to assess overall farms efficiencies. As a second stage, the effect of the WUAs’ financial and technical performance on these efficiency scores will be assessed using econometric regressions.
- Production Functions and Efficiency: Some Theoretical Issues
This section discusses production functions and some related concepts which form the basis of measuring the efficiency of farms. We explain the basic concepts of technical, allocative and economic efficiency. The measurement of efficiency was first proposed by Farrell (1957). The failure to produce the maximum output from a given input mix at minimum cost results in inefficiency. Inefficiency is explained by, inter alia, restricted access to technology, a lack of knowledge, restricted access to extension services, inappropriate scale of production and sub-optimal allocation of resources. The efficiency of a farm consists of two components: technical and allocative efficiency. Technical efficiency concerns the ability of a farm to produce maximum output from a given set of inputs using existing technology; allocative efficiency reflects the ability of a farm to choose the inputs in optimal proportions, given their respective prices; and a combination of these two measures provides a measure of economic efficiency. Thus economic efficiency concerns the ability of a farm to produce output at minimum cost to obtain this minimum cost, the farm uses inputs in an efficient manner (technical efficiency) and chooses a cost-minimizing combination of inputs, given input prices and marginal productivities.
- Production Functions
In microeconomic theory, the production function explains the technical or physical relationship between output and inputs. Specifically it shows the maximum output obtainable from a given set of inputs. Inputs are rates of resource use and output is the rate of production over a specific time period. Let (x1,x2...xn) denote the inputs used in the production of output y; the production function can be written as:
(1)
The production combinations which maximize y for given x or minimize x for given y are technically efficient combinations constitute the boundary to the production set Q = (y,x). Thus the production function y = f(x) is the set of technically efficient combinations, and all technically inefficient combinations belong to the interior of the production set.
An isoquant or production indifference curve is defined as the locus of all the technical efficient combinations of inputs which produce the same output. It shows the rate at which inputs are substituted in production holding output constant. For simplicity consider the two variable production function:
(2)
The equation of an isoquant is obtained by the production function (2) when output is held constant at say y0:
(3)
This represents the isoquant which displays all combinations of inputs that can be used to produce output y. The slope of the isoquant at any point is derived by differentiating (4.4) implicitly with respect to one of the inputs, say x 1 . This yields:
(4)
The negative of the slope of an isoquant is the marginal rate of technical substitution (MRTS) which measures the rate at which inputs can be substituted, keeping output constant.
The isocost line shows the rate at which inputs are exchanged in the market (their relative prices). It is the locus of all combinations of inputs that can be purchased with a given cost outlay, that is, the isocost line is the locus of input combinations that entails the same total cost C0:
(5)
where P1 and P2 are the input prices of x1 and x2 and its slope is found by differentiating the isocost line:
(6)
Which is the negative of the ratio of the input prices.
- Choice of Cost Minimizing Input Mix
We explain the problem of finding the least-cost input combination that the farm chooses for the production of a specified level of output y0. The choice of a cost-minimizing input combination deals with the issue of how cost can be minimized? We assume that output and the price of the inputs are given.
Cost minimization requires the tangency of the given isoquant with the lowest possible isocost line.
The farm minimizes its costs by using input combination (x1 ,x2 ) determined by the tangency point of the given isoquant y0 with the isocost line . The cost-minimizing input combination is obtained at a point where the slopes of the isoquant and the isocost line are equal.
(7)
Obtaining the cost-minimizing input vector ensures allocative efficiency.
- Measures of Efficiency
Defining Efficiency
The term “efficiency” implies the success with which a farm best utilizes its available resources to produce maximum levels of potential outputs (Dinc et al., 1998). A farm is efficient if and only if it is not possible to increase output (decrease inputs) without more inputs use (without decreasing output) (Cooper et al., 1995). Failure to obtain this potential maximum output results in inefficiency.
The concept of productive efficiency was first introduced by Michael Farrell (1957) who argued that there were two components of efficiency: technical efficiency (TE) and allocative efficiency (AE).
The production process of a farm may reflect technical inefficiency, allocative inefficiency or both. A farm is technically efficient if it produces a maximum output, given the amount of inputs and technology.
Allocative inefficiency arises if farms fail in allocating inputs which minimize the cost of producing a given output, given relative input prices. This results from not allocating inputs in the most efficient manner. In particular, a farm is said to be allocatively inefficient if the marginal rate of technical substitution between any two inputs is not equal to the corresponding ratio of input prices.
According to Farrell’s methodology, economic efficiency (EE) is equal to the product of TE and AE that reflects the ability of a farm to produce output at minimum cost. Thus, EE is defined as the capacity of a firm to produce a predetermined quantity of output at minimum cost for a given level of technology (Farrell 1957; Kopp and Diewert 1982).
In Figure 1, it is assumed that there are two inputs (X1 and X2) used by a firm to produce a single output (Y) with assumption of constant returns to scale. The II’ curve represents the isoquant of fully efficient firms, and could be used to measure TE. If the firm employs amount inputs at point R to produce a unit of output, the technical inefficiency of that firm could be measured by the distance RS. This is the proportion by which the use of inputs could be reduced without a decrease in output. This is expressed in percentage terms by the ratio SR/OR, which stands for the percentage by which all inputs need to be reduced to gain production which is technically efficient. The TE of a firm is measured by the ratio: TE = OS/OR. If a firm has TE equal to 1, it is technically efficient. The firm is technically inefficient if its TE value is less than 1. is equal to 1, the firm produces with full technical efficiency. At point S the firm could gain full technical efficiency because point S lies in the efficient production indifference curve.
|
Figure 1 : Technical, Allocative and Economic Efficiency
|
If input prices are known, we can calculate AE. I I’ is isoquant and CC’ is the isocost where the latter is tangent to the former at point E. The marginal rate of technical substitution between the two inputs is equal to input price ratio at this point. At E the cost of producing a given level of output is the minimum as it represents the most efficient allocation of inputs. For output quantity produced at point S, the best use of inputs is at point E because it represents the minimum cost. The AE of the firm can be defined as: AE = OT/OS. The firm is technically as well as allocatively efficient at point E.
The range from T to S shows the reduction in cost of production provided production were to take place at allocatively and technically efficient point E rather than point S which is technically efficient but allocatively inefficient. The firm would be fully allocatively efficient if its AE value is 1 and allocatively inefficient it its AE value is less than 1. The economic efficiency is a combination of technical and allocative efficincy and can be obtained by multiplying TE and AE.
EE = TE * AE = OS/OR * OT/OS = OT/OR ( 8)
The index of EE also varies between 0 and 1 where the latter implies that the firm is economically efficient. If the value of EE is less than 1 then the firm is economically inefficient. The distance from R to T also represents the cost reduction in production if a firm produces at point T with technical and allocative efficiency, instead of at point R with technical and allocative inefficiency. Economic efficiency is a combination of technical and allocative efficiency.
Efficiency estimation
The efficiency measures proposed by Farrell assume a known production function for the fully efficient DMU. The production function of a DMU is generally unknown in practice, and relative efficiencies must be measured from the sample data available. Two approaches are used to estimate relative efficiency indexes: the parametric or stochastic frontier production approach (SFA) and the nonparametric or data envelopment analysis approach (DEA) (Coelli, 1995). The SFA assumes a functional relationship between outputs and inputs and uses statistical techniques to estimate parameters for the function. It incorporates an error composed of two additive components: a symmetric component that accounts for statistical noise associated with data measurement errors and a non-negative component that measures inefficiency in production (Coelli, 1995).
In contrast, the nonparametric approach Data Envelopment Analysis ( DEA) uses linear programming methods to construct a piecewise frontier of the data. Because it is nonparametric, DEA does not require any assumptions to be made about functional form or distribution type. It is thus less sensitive to misspecification relative to SFA. However, the deterministic nature of DEA means all deviations from the frontier are attributed to inefficiency. It is therefore subject to statistical noises resulting from data measurement errors (Coelli, 1995). The choice of which method to use appears to be arbitrary, as is pointed out by Dhungana et al., (2004). We chose the DEA approach in this study, since it imposes no a priori parametric restriction on the underlying technology (Chavas and Aliber, 1993; Fletschner and Zepeda, 2002).
Technical, Economic, and Allocative Efficiency DEA Model Specifications
When using DEA we may make choice between Constant Returns to Scale (CRS) and Variable Returns to Scale (VRS) assumptions . CRS assumes that there is no significant relationship between the efficiency and the scale of operation, thus assuming that large farms are just as efficient as the small ones in converting inputs to outputs. In our case most farms have small size and the area disparity is not important, since the option of CRS will be selected in this study.
Under the non-parametric approach (DEA), to estimate the production frontier, we consider the “input oriented” model, according to colli (1996) and we have in the model presented here : n farms (i=1,……n), each producing M outputs ymn (m=1,…M) by using K different inputs xkn(k=1,…..K), each farm becoming the reference unit. For the ith firm we have vectors xi (K*1) and yi (M*1). For the entire data set, therefore, we have a K*N input matrix X and M*N output matrix Y. The technical efficiency (TE) measure is obtained by solving the following DEA model
Sc
(9)
Where θ is a variable representing the efficiency of the Reference Farm i and hence the percentage of reduction to which each input must be subjected to reach the production frontier. λ is a vector of (k*1) elements representing the influence of each farm in determining the efficiency of the ith farm. λk is a vector of k elements representing the influence of each DMU in determining the efficiency of the the ith farm.
Formally, the displacement along the isoquant towards the point where costs are minimized resulting in minimizing the following cost function:
Sc
(10)
where :
w’i : the inputs prices vector used by the ith farm
x*i : the minimal cost calculated by the model taking in account the prices w’i and the production level yi of the ith farm.
is a vector of (k*1) elements presenting the weighting coefficient of inputs.
The total or overall economic efficiency (EE) index for the ith firm is then computed as
(11)
which is the ratio of the minimum cost to the observed cost). The allocative efficiency (AE) index, can be derived from Eq. (8) :
(12)
It should be noted that Eq. (10) also accounts for input slacks not captured by Eq. (9) above. Following Ferrier and Lovell (1990) this procedure attributes any input slacks to allocative inefficiency on the grounds that slack reflects an inappropriate input mix. The CRS or 'overall' (TECRS) measure can be decomposed into its 'pure' TE and scale efficiency components by solving a variable returns to scale (VRS) DEA model, which is obtained by imposing the additional constraint, on Eq. (9) (Banker et al., 1984). Let θiVRS denote the TE index of the ith Farm under variable returns to scale (TEVRS), then the technically efficient cost of production of the ith farm under VRS DEA is equal to W’i .θi VRS .Xi.
- Determining factors affecting efficiency
Many researchers have analysed the determinants of the productive efficiency of farms by liking them to farms-specifics factors. In our case we examine the effects of WUAs’ characteristics and performances on farms economic efficiency.
The WUA is formed by many adherents whose supposed to collectively share and mange the same resource. This organisation constitutes a form of institutional arrangement for water resource management. The success (or the failure) of this arrangement will be reflected by the active participation of the WUA’s members. The WUA’s performance is in turn supposed to affect farms’ economic efficiency. Most importantly two major WUAs’ factors can affect the farms efficiency and so their participation: the Budget deficit and the water turn time.
Budgetary deficit (DEFICITBUD): is the difference between the annual total production cost (fixed cost + operating cost) and the revenue generated by water sales at the end of year. It indicates the level of financial performance and arrangement’s autonomy.
It is assumed that this autonomy gives WUA some flexibility of action which enables it to timely intervention and improves both its services and its technical performance which affect the farms participation and efficiency.
The water turn time (TURN): This variable is expressed in number of days. Water turn is the main constraint to farmer who must juggle the crops’ needs, the equipments and time allowed for irrigation. This specific variable can be a main constraint to an agronomic optimisation of the crops’ needs and it explains the water shortage too. It’s essentially a function of the drilling flow, the number of WUA member and the irrigated area served by the WUA. More irrigators are satisfied when drilling’s flow is high, while high water turns are essentially due to the number of WUA. The water turn explains a form of the WUA’s technical performance. In fact, when the network efficiency is high will better satisfy the water demand which can reduce the water turn and eventually the conflicts around the resource.
After calculating the efficiency measures, the next step is to identify the arrangements on the farms’ efficiency, something commonly done by estimating a second-stage relationship between the efficiency measures and suspected correlates of efficiency (Binam et al., 2003). Since the efficiency parameters vary between 0 and 1, they are censored variables and thus a Tobit model needs to be used.
=
(13)
Where θi are technical, allocative and economic efficiency used as dependant variables. θ* : is the value of an artificial variable (unobservable) that is related to explanatory variables (Xi) as the following relationship:
Where: ԑi error term and β are parameters to be estimated.
The estimation of the Tobit model is based on maximum likelihood procedures. For Tobit estimates to be consistent, it is necessary that residuals are normally distributed (Holden, 2004).
- Data and empirical procedures
- Data
This research was conducted at the Nadhour region which is located at the southern part of the governorate of Zaghouan in the center of Tunisia. It is characterized by a semi-arid climate. The average annual rainfall is 400 mm. The agricultural area of Nadhour is around 38,200 ha. Nadhour region accounts about 1925 farms, 60 % of them with an area less than 5 ha and 28 % with a farm size ranging between 5 and 10 ha. The irrigated systems of the region were installed since 1980, and the total irrigated area is about 3050 ha. Most irrigated areas are planted with summer crop (watermelon, pepper, melon, season tomato…). The water resources are about 14 million m3. Two thirds of these resources are groundwater.Water demand management is ensured by 34 WUAs. These WUAs ensure thesale of water to users and networks maintenance. The pricing method most used is the volumetric pricing. The irrigation water rate varies from 0.085 to 0.13 TD/m3. The problem of water availability usually arises during peak periods corresponding to the months of May, June, July, August, and September. Data is collected through a survey on 75 farmers representing 38 % of the total number of members from 6 WUAs during 2011 ( Table 1). The total perimeters areas served by WUAs ranges between 50 and 70 ha. These WUAs are marked by a low rate of exploitation. The latter is between 40 % at the perimeters Chaalil Sud and Naffet and 67% at the perimeter Nadhour 2. The applied price is based on the calculation of operating costs incurred by the WUA to pump and distribute irrigation water to different members. These expenses mainly cover the costs of energy, labor, and maintenance. It forms the operational cost of irrigation water pumped and distributed. This rate covers operational costs with a slight margin of no more than 0.01 TD/m3. It varies from one to another WUA. The lowest rate is recorded at the WUA Zwagha 2 which is 0.09 TD/m3 against a rate of 0.13 TD/m3 at theWUA Chaalil Sud. The average price at the level of 6 WUAs is approximately 0.103 TD/m3 (Table 1). Water consumption per hectare is calculated as the total consumption of perimeter / (the total area of the perimeter × exploitation rate). In average this value is about 4082 m3. This varies across the considered areas. It is noted that the lower consumption is recorded at the WUA Nadhour 2 which is about 3458 m3/ha against the highest consumption level recorded at WUA Chaalil Sud which amounts to 6000 m3/ha. This disparity shows a close correlation between the consumption and exploitation rate that is closely linked with the intensification rate.
Table 1 : WUAs characterization |
|||||||
WUA |
I |
II |
III |
IV |
V |
VI |
Total |
Members Number |
40 |
19 |
14 |
18 |
33 |
35 |
183 |
perimeter Area |
50 |
50 |
70 |
50 |
60 |
70 |
380 |
Average area/farm |
1.25 |
2.6 |
5 |
2.8 |
1.8 |
2.0 |
2.08 |
Exploitation rate |
40% |
50% |
57% |
40% |
67% |
63% |
53% |
Water price |
0.1 |
0.1 |
0.09 |
0.13 |
0.12 |
0.1 |
0.103 |
Flow |
25 |
15 |
45 |
15 |
20 |
25 |
24 |
Consumption/Ha |
3600 |
5000 |
4261 |
6000 |
3458 |
3900 |
4082 |
Nb of investigated farmers |
20 |
8 |
10 |
11 |
15 |
11 |
75 |
% of total number of members |
50% |
42% |
71% |
61% |
45% |
31% |
38% |
I: Naffet; II: Zwagha1; III: Zwagha2; IV: Chaalel Sud; V: Nadhour2 ; VI : Nadhour3.
|
Land use
The analysis of land use at our research sites shows that irrigated areas are mainly cropped by watermelon ranging between 21% at Zouagha 2 to 57% at Naffet. followed by tomatos that varies from 10 to 40%. Pepper comes third given its importance and it occupies between 12 and 37% of cultivated land.
The importance of cereals is also variable from one perimeter to another where it does not exceed 10% in areas at Zwagha1 and Nadour2 to 23 and 48% respectively in the perimeters Nadhour 3 and chaalel sud.
For the legumes and despite their importance in crop rotations. they are almost absent in the existing farming systems since they do not appear in the perimeters of Zwagha1 and chaalil sud and do not exceed 12% in the perimeter of Nadhour 2.
The analysis of the cropping calendar shows that crops grown in the dry season of the year are long cycle and spread over the whole season. These crops are also very demanding in water which will increase the total water demand for irrigation. Indeed. during the season stretching from April to September, farmers used to grow watermelon. tomato. pepper and cucumber which are all high water consuming crops. These practices will influence the quality of the management of supply and demand for water at the WUA level. The increase in demand leads to conflicts between farmers both for the water turn than the amount requested.
- Description of variables used to estimate the production frontier
Results of our investigations show that the main inputs used are the expenses for seeds and plants. mechanization. fertilization and pesticides treatments. All of these expenses are aggregated into a single variable that is the Capital (K). The other inputs taken into account are the irrigable area (SAUIR) in Ha. The amount of water consumed (Water) in m3 and labor (L) in days.
The output of the used technology is the value of the farm production (Y) in TND. It is determined by the aggregation of the productions values of all irrigated activities. Basic statistics regarding the selected WUAs are shown in Table 2.
- Results
- efficiency calculations
DEA models were estimated using the program. DEAP 2.0 (Coelli. 1996). The technical. allocative and economic efficiency measures estimated from the DEA approach and their frequency distributions are presented in Table 3 to table 5.
Table 3 : Farms’ technical efficiency distribution/WUA
|
|||||||
WUA |
I |
II |
III |
IV |
V |
VI |
Total |
Average |
0.68 |
0.81 |
0.56 |
0.70 |
0.75 |
0.62 |
0.68 |
Standard deviation |
0.13 |
0.20 |
0.25 |
0.22 |
0.27 |
0.23 |
0.23 |
Min |
0.46 |
0.29 |
0.20 |
0.30 |
0.25 |
0.24 |
0.20 |
Max |
0.87 |
1.00 |
1.00 |
1.00 |
1.00 |
1.00 |
1.00 |
% of farms with TE > 0.68 |
50% |
90% |
36% |
60% |
64% |
40% |
55% |
|
|
|
|
|
|
|
|
The resulting technical efficiency indexes are presented in Table 3. Thus these scores range from 0.20 to 1. The farms’ average technical efficiency varie from 0.56 at Chaalil Sud WUA to 0.81 at Zwagha 2 WUA. The average efficiency provides information about the potential resource saving that could be achieved while maintaining the same output level. The results mean that a 32% decrease in all inputs is possible with the present state of technology and unchanged outputs. or the same level of output can be reached by only using 68% of the used inputs, if technical inefficiency is completely removed. In general, more than 55% of farms have efficiency greater than 0.68, this proportion varies with in the perimeters. It has a value of 36% in Chaalil Sud WUA and a value of 90% at Zwagha2. In fact the farms of Zwagha 2 are the most efficient with an average of 81%. Moreover, 90% of farms within this WUA have a technical efficiency greater than 68%, followed by those of Nadhour3 with an average TE of 75% and 64% of farms having a TE greater than the overall average TE. This distribution shows the effect of the institutional arrangement on the farms’ efficiencies.
The results of the allocative efficiency are summerised in the table 4. The mean of allocative efficiency measure estimated from the DEA frontier is 71%. This average ranges from 0.65 at Chaalil Sud WUA to 0.81at Zawagha 2 WUA, showing a potential reduction of the cost while maintaining constant the production level by 29%. The portion of farms with an AE greater than the average is of 51%.
Table 4 : Farms’ Allocative efficiency distribution/WUA
|
|||||||
WUA |
I |
II |
III |
IV |
V |
VI |
Total |
Average |
0.71 |
0.81 |
0.65 |
0.66 |
0.72 |
0.73 |
0.71 |
Standard deviation |
0.06 |
0.09 |
0.15 |
0.16 |
0.18 |
0.11 |
0.14 |
Min |
0.64 |
0.66 |
0.46 |
0.25 |
0.24 |
0.56 |
0.24 |
Max |
0.83 |
0.94 |
0.87 |
0.83 |
0.92 |
1.00 |
1.00 |
% of farms with AE > 0.71 |
38% |
90% |
36% |
33% |
73% |
45% |
51% |
|
|
|
|
|
|
|
|
The Zwagha2 WUA is ranked at the first place with 90% of farms that their AE are greater than 0.71. More than 55% of the farms located in the other WUA have an AE under the average.
The mean EE score across farms is 0.48. and ranges from a minimum of 0.07 to a maximum of 1 (table6). These results indicate farms are economically inefficient on average, and that the total cost of production for each farm can be reduced on average by approximately 52% to achieve the same level of output.
Table 5 : Farms’ economic efficiency distribution/WUA
|
|||||||
WUA |
I |
II |
III |
IV |
V |
VI |
Total |
Average |
0.48 |
0.65 |
0.34 |
0.47 |
0.53 |
0.46 |
0.48 |
Standard deviation |
0.08 |
0.19 |
0.13 |
0.18 |
0.25 |
0.20 |
0.20 |
Min |
0.32 |
0.25 |
0.15 |
0.07 |
0.18 |
0.21 |
0.07 |
Max |
0.58 |
0.93 |
0.55 |
0.70 |
0.89 |
1.00 |
1.00 |
% EE>0.48 |
75% |
90% |
9% |
53% |
55% |
40% |
51% |
|
|
|
|
|
|
|
|
The EE average observed across the WUAs ranges from 0.34 at Chaalil sud to 0.65 Zawagha2. The proportion of farms with EE above the average is 51%. This classification puts in first place the WUA of Zwagha 2 with 90% of farms followed by Zwagha1 with 75% of farms. While 91% of the Chaalil sud WUA farms have EE scores under the Average followed by those of Naffet with an percentage of 60%. This classification allows us to identify the arrangements’ (WUA) effects on the Farms’ performance. This effect is expressed by the proportion of farms that have the highest efficiency scores. this is illustrated by table 6 in which we classified the farms into quartiles holding from less to more efficient.
The table 6 indicates that within the first quartile(Total number of farms is 75)holding the 19 less efficient farms (0.07<EE<0.32) there are 45% of farms belonging to Chaalel Sud WUA, 35%of farms belonging to Naffet WUA are also in this quartile, against only 10 and 13% of farms belonging respectively to Zwagha2 and zwagha1.It looks indeed that any arrangements’ performance improvement implies improving the farms’ performance.
Table 6 : distribution of farms /quartile
|
||||
WUA |
1st quartile |
2nd quartile |
3thquartile |
4th quartile |
(the less efficient farms) |
(the most efficient Farms) |
|||
Chaalil-Sud |
45% |
45% |
9% |
0% |
Nadhour2 |
13% |
33% |
33% |
20% |
Nadhour3 |
27% |
18% |
9% |
45% |
Naffet |
35% |
25% |
20% |
20% |
Zouagha1 |
13% |
25% |
63% |
0% |
Zouagha2 |
10% |
0% |
30% |
60% |
Total |
25% |
25% |
25% |
24% |
|
|
|
|
|
This relation will be clearer by regressing the efficiencies’ scores by the explanatory variables of this performance related to WUAs as described in the next section.
- Determinants of farmer’s efficiencies
The analysis of farms’ performances has shown significant differences between productive efficiencies in different perimeters. This difference is due to the variability of WUAs’ effects. Two explanatory variables are retained that explain the arrangement performance: the budget deficit and the water turn time as described above. The results of Tobit model by maximum likelihood procedure are shown in Table 7.
Table 7 : results of Tobit model
|
||||||
|
TE |
AE |
EE |
|||
Variables |
Coefficients |
P |
Coefficients |
P |
Coefficients |
P |
DEFICITBUD |
-0.014 (0.003) |
0.000 |
-0.003 (0.001) |
0.09 |
-0.011 (0.022) |
0.000 |
TURN |
-0.045 (0.012) |
0.000 |
-0.017 (0.007) |
0.023 |
-0.045 (0.090) |
0.000 |
Sigma |
0.215 (0.018) |
|
0.135 (0.011) |
|
0.163 (0.013) |
|
LR ch1(2) |
22.66 |
|
5.9 |
|
30.28 |
|
Prob> chi2 |
0.000 |
|
0.05 |
|
0.000 |
|
Log likelihood |
0.45 |
|
39 .22 |
|
25.47 |
|
N |
75 |
|
75 |
|
75 |
|
|
|
|
|
|
|
|
We note that the likelihood ratio (LR) is statistically significant at 1%. Thus the model is generally acceptable. The estimation results obtained allow us to note that the explanatory variables are significant at the 1% for technical and economic efficiency and the threshold of 5% and 10% for estimating allocative efficiency. So, the variable budget deficit (DEFICITBUD) which expresses the financial performance of the GDA has a negative impact on technical, allocative and economic efficiency and it’s statistically significant at the 5% threshold, revealing that when the deficit increases farm economic performance decreases. Indeed, its negative coefficient of -0.014 for TE indicates that reducing the budget deficit by one unit (in 1000 TND) causes an improvement in the technical efficiency of farms by 1.4%. Reducing the budget deficit has also a positive effect on the AE with a coefficient of -0.003. The coefficient of this variable in the EE estimation equation shows that reducing the budget deficit by one unit leads to a marginal increase in EE by 1.1%.In our case the average economic efficiency is 0.48 and the average budget deficit is 17.5, indicating that the potential improvement in economic performance is 17.5 *1.1 = 19.25% to move from an average EE level of 0.48 to 0.67 level. So to reduce the deficit it is necessary to improve the recovery rate that is negatively correlated with the budget deficit involving a high recovery rate and leading to an increase in the farms’ efficiency. The increase of water fees may in turn results in performance improvement of farms. This will improve the financial situation of the WUA and consequently its performance and autonomy. This autonomy allows the WUA to intervene at the appropriate time about the network maintenance and payment of its obligations to various creditors (Electrical company, state, subcontractors ...).These various operations promote the services provided by the WUA to members which stabilizes the supply and harmony between the different actors and gives more certainty and confidence to users who will take appropriate decisions and plans production activities with serenity.
The table 8 shows another important result. It’s the negative effect of the water turn on the farms’ efficiency. The statistically significant relationship at the level of 1% between economic efficiency and the water turn expressed by the coefficient of -0.045 indicates that when the water turn increases by 1 day the economic efficiency decreases by 4.5%. The water turn has also the same effect on technical efficiency and statistically significant at 1% level. Results show that an increase in the water turn time by one day reduces the allocative efficiency by 1.7%.This explains the effect of WUAs technical performance on those of farms. Thus the WUA’s members must make arrangements to establish the shortest water turn as possible by acting mainly on the distribution network efficiency, by minimizing water losses at the network level and by coordinating on the temporal distribution of crops as well as on area cultivated by each farm to ease the pressure on the resource. The control of water loss increases the flow and satisfies a maximum number of users per unit of time. This result is a very good indicator of the WUA’s performance to control the resource and to better meet the farms’ needs. This is also showing that farmers will be winners by ensuring the improvement of the water turn time, especially the reduction in the loss of the resource will reduce also the cost per m3 of water distributed. So farmers will participate with more determination to the success of collective action when they see that the WUA is ensuring their satisfaction, compressing the cost and consequently reducing the water price. The positive correlation between the water turn and the number of members (expressing the size of the WUA) implies the negative influence of the size of WUA on farms efficiency which means that farmers belonging to larger WUA are also the less efficient. This implies that when collective action is conducted by a small group of persons, the share of each participant will be larger and the potential benefits of participation will be more important which encourages farmers to contribute positively to the action. Moreover, the limited number of adherents reduces the monitoring costs and free rider effects of someone will be easier to control.
- Conclusion
This paper analyses technical. allocative and economic efficiency for a sample of 75 farms belonging to 6 WUAs in the centre of Tunisia using the nonparametric frontier approach (DEA). The effect of various factors on the efficiency levels is examined by estimating a regression model where efficiency of farms’ production are expressed as a function of various WUA specific factors. The low water price practiced does not allow the fixed cost recovery of water production and induce budget deficit of the WUAs. This will affect the services delivered by these arrangements as technique which will affect in turn the farms’ productivity. Results show the average technical, allocative and economic efficiencies under constant return to scale are: 68%, 71% and 48% respectively. The results reveal too, substantial production inefficiencies among farms and hence considerable potential for enhancing profitability by reducing costs through improved efficiency. On average, by operating at full economic efficiency levels the sample producers would be able to reduce their cost by 52% by reducing the WUAs’ budget deficits and Water turn time. Since, the analysis of WUAs’specific factors shows that budget deficit has a negative and significant effect on efficiency levels, suggesting that cost inefficiency can be reduced by increasing water price to cover the fixed cost. Results also show a negative relationship between a water turn time and farms ‘economic efficiencies. In general improving institutional arrangements leads to improve farms’ performances.
- References
Abdelhafidh H. and M.S. Bachta. 2016. Groundwater pricing for farms and water user association sustainability. Arabian Journal of Geosciences. (2016). 9-525.
Al Atiri R. 2005. WP2 Wademed on economic instruments for the modernization of irrigated agriculture. Analysis of water policies. Case of Tunisia. Sousse. Tunisia. in November 2005 “(in French)”.
Bachta M.S.. Ghersi G., 2004. Agriculture and Food in the Mediterranean: the challenges of globalization. Montpellier.France: Editions Karthala /CHIHEAM/IRESA (in French).
Bachta M.S.. Zaibet L., 2007. The institutional innovations as adaptations to the changing environment of irrigated areas: the case of Tunisia. In: Bouarfa S. et al. (eds.). The future of irrigated agriculture in the Mediterranean. New institutional arrangements for managing water demand. Proceedings of the seminar Wademed. Cahors. France. 6-7 November 2006. CIRAD. Montpellier. France (in French).
Banker. R.D.. Chames. A.. Cooper. W.W.. 1984. Some models for estimating technical and scale efficiencies in data envelopment analysis. Manage. Sci. 30. 1078-1092.
Binam J.N.. Sylla K.. Diarra I.. Nyambi G.. 2003. Factors affecting technical efficiency among coffee farmers in Cote d’Ivoire: evidence from the Centre West Region. R&D Management. 15: 66-76.
Coelli. O.J. 1995. Recent Development in Frontier Estimation and Efficiency Measurement. Austalian. Journal of Agricultural Economics. 39. pp. 219–245.
Coelli. T.J.. 1996. A guide to DEAP Version 2.0: a data envelopment analysis (computer) program. Center for Efficiency and Productivity Analysis (CEPA) Working Paper 96/ 08. Department of Econometrics. University of New England. Armidale. Australia.
Cooper. W.W. S.C. Kumbhakar. R.M. Thrall and X.L. Yu. 1995. DEA and Stochastic Frontier Analyses of the 1978 Chinese Economic-Reforms. Socio-Economic Planning Sciences. 29. 85-112
Dhungana. B.R.. P.L. Nuthall. and G.V. Nartea. 2004. “Measuring the Economic Inefficiency of Nepalese Rice Farms using Data Envelopment Analysis.” The Australian Journal of Agricultural and Resource Economics 48(2004):347-369.
Dinc. M.. K.E. Haynes. R.R. Stough. and S. Yilmaz. 1998. Regional Universal Telecommunication Service Provisions in the US: Efficiency versus Penetration. Telecommunication Policy. 22. 541-553.
Farrell. M.J.. 1957. The Measurement of Productive Efficiency. Journal of Royal Statistical Society. ACXX. part 3. 153-290.
Ferrier. G.D.. Lovell. C.A.K.. 1990. Measuring cost efficiency in banking: econometric and linear programming evidence. J. Econom. 46. 229-245.
Fletschner. D.K. and L. Zepeda. 2002. “Efficiency of Small Landholders in Eastern Paraguay.” Journal of Agricultural and Resource Economics 27(2002):554-572.
Kopp. R. J.. and W. E. Diewert (1982). The Decomposition of Frontier Cost Functions Deviations into Measures of Technical and Allocative Efficiency. Journal of Econometrics. 19 (2-3). pp. 319–31.
MAHDHI N.. SGHAIER M. and SMIDA Z.. 2014. Efficiency of the irrigation water user association in the Zeuss-Koutine region. south-eastern Tunisia. NEW MEDIT N. 2/2014. 47-55.