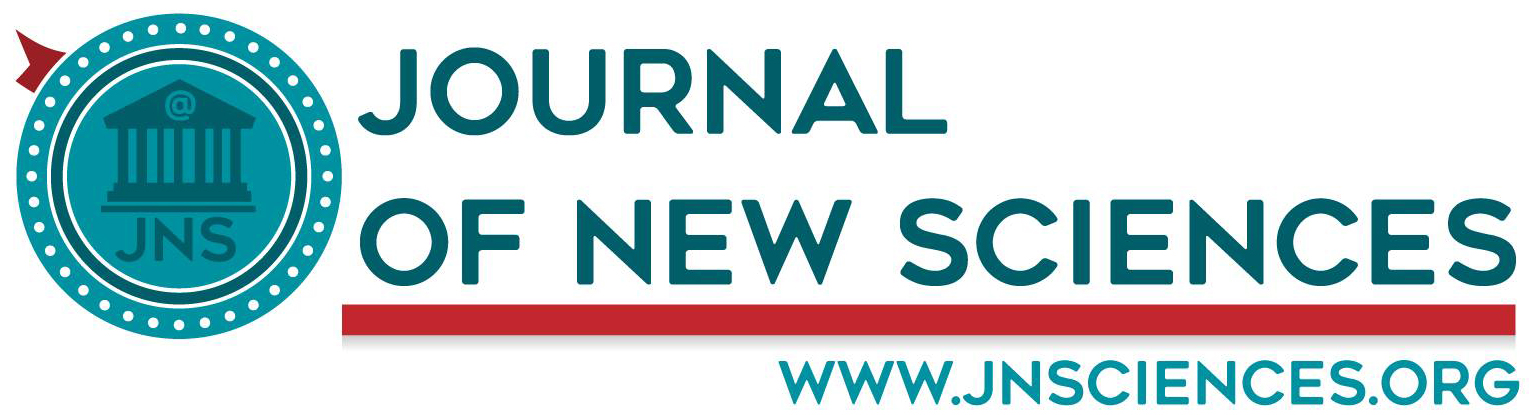
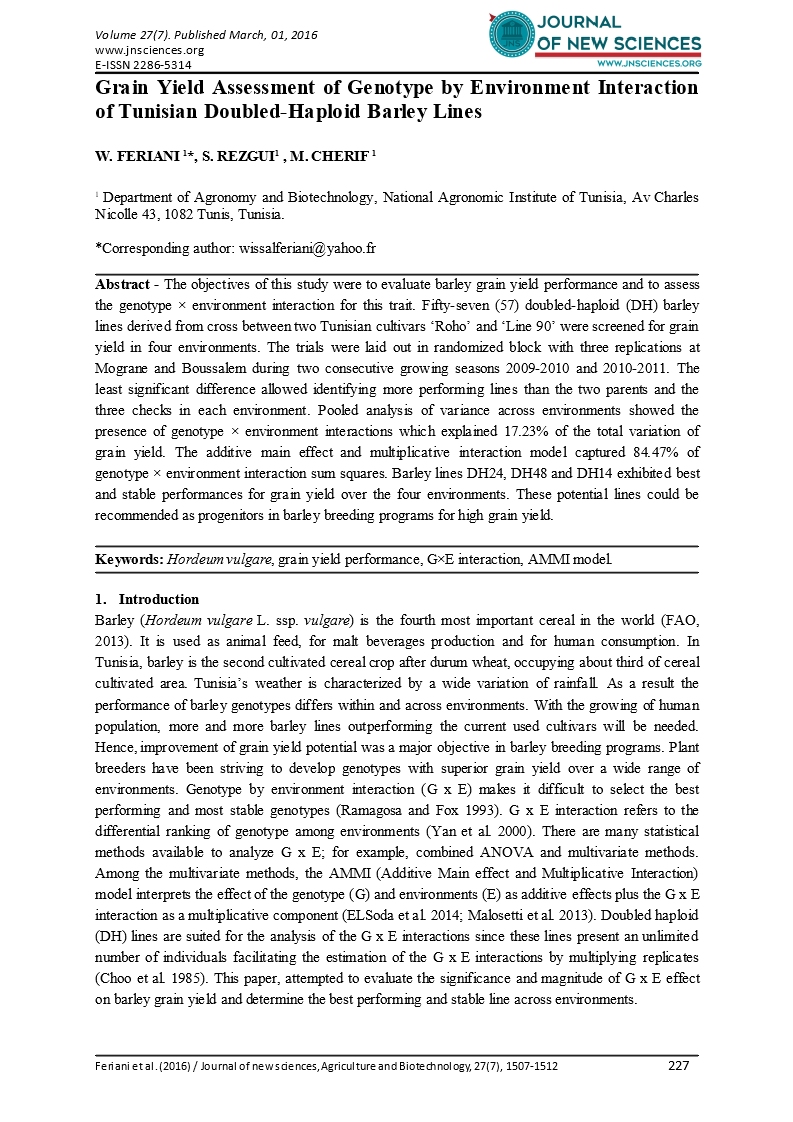
- Category: Volume 27
- Hits: 5682
Grain Yield Assessment of Genotype by Environment Interaction of Tunisian Doubled-Haploid Barley Lines
W. Feriani 1*
S. Rezgui1
M. Cherif 1
1 Department of Agronomy and Biotechnology, National Agronomic Institute of Tunisia, Av Charles Nicolle 43, 1082 Tunis, Tunisia.
Abstract - The objectives of this study were to evaluate barley grain yield performance and to assess the genotype × environment interaction for this trait. Fifty-seven (57) doubled-haploid (DH) barley lines derived from cross between two Tunisian cultivars ‘Roho’ and ‘Line 90’ were screened for grain yield in four environments. The trials were laid out in randomized block with three replications at Mograne and Boussalem during two consecutive growing seasons 2009-2010 and 2010-2011. The least significant difference allowed identifying more performing lines than the two parents and the three checks in each environment. Pooled analysis of variance across environments showed the presence of genotype × environment interactions which explained 17.23% of the total variation of grain yield. The additive main effect and multiplicative interaction model captured 84.47% of genotype × environment interaction sum squares. Barley lines DH24, DH48 and DH14 exhibited best and stable performances for grain yield over the four environments. These potential lines could be recommended as progenitors in barley breeding programs for high grain yield.
Keywords: Hordeum vulgare, grain yield performance, G×E interaction, AMMI model.
-
Introduction
Barley (Hordeum vulgare L. ssp. vulgare) is the fourth most important cereal in the world (FAO, 2013). It is used as animal feed, for malt beverages production and for human consumption. In Tunisia, barley is the second cultivated cereal crop after durum wheat, occupying about third of cereal cultivated area. Tunisia’s weather is characterized by a wide variation of rainfall. As a result the performance of barley genotypes differs within and across environments. With the growing of human population, more and more barley lines outperforming the current used cultivars will be needed. Hence, improvement of grain yield potential was a major objective in barley breeding programs. Plant breeders have been striving to develop genotypes with superior grain yield over a wide range of environments. Genotype by environment interaction (G x E) makes it difficult to select the best performing and most stable genotypes (Ramagosa and Fox 1993). G x E interaction refers to the differential ranking of genotype among environments (Yan et al. 2000). There are many statistical methods available to analyze G x E; for example, combined ANOVA and multivariate methods. Among the multivariate methods, the AMMI (Additive Main effect and Multiplicative Interaction) model interprets the effect of the genotype (G) and environments (E) as additive effects plus the G x E interaction as a multiplicative component (ELSoda et al. 2014; Malosetti et al. 2013). Doubled haploid (DH) lines are suited for the analysis of the G x E interactions since these lines present an unlimited number of individuals facilitating the estimation of the G x E interactions by multiplying replicates (Choo et al. 1985). This paper, attempted to evaluate the significance and magnitude of G x E effect on barley grain yield and determine the best performing and stable line across environments.
-
Materials and methods
-
Plant material
Plant material used in this study consisted of fifty-seven DH barley lines obtained at Florimond Desprez (France), using anther culture and the Hordeum bulbosum method (Pickering and Devaux, 2005). These DH lines were developed from F1 plants of the cross between the Tunisian cultivar 'Roho' and the local line '90' which was carried out at INAT (Institut National Agronomique de Tunisie). Three barley genotypes ‘Martin’, ‘Manel’ and ‘Rihane’ were added to the present population as checks.
-
Field experiments
The experiments were conducted during two growing seasons 2009-2010 and 2010-2011 at the farm of ESA - Mograne (36°25‘59"N 10°5‘59"W) (Altitude 138 m), in the district of Zaghouan in north western Tunisia about 60 km south of the capital; and at the experimental station (Institut National des Grandes Cultures) of Boussalem (36°36‘34"N 8°58‘17"W) (Altitude 127 m) in the district of Jendouba in the extreme north west of Tunisia.
The trials were laid down in a randomized block design with three replications. Barley lines were sown on 12 November 2009 and on 30 November 2010 at Mograne. The sowing dates at Boussalem site were on 20 November 2009 and 23 November 2010. Each genotype was sown in two rows of two meters length with 25 cm inter-rows spacing and 5cm between plants spacing. Grain yield (GY) was assessed as the total grain weight of two rows measured after harvest, and expressed in grams per meter square (g/m2).
-
Statistical analysis
In the analysis, each combination of location × growing season was treated as an environment (E), making a total of four environments (Mograne (Mg) 2009-2010, Boussalem (BS) 2009-2010, Mograne (Mg) 2010-2011 and Boussalem (BS) 2010-2011).
Grain yield (GY) data was first analyzed using PROC ANOVA of SAS (SAS Institute, 1988) separately for each environment to assess variation within barley genotypes according to the following model:
Yij = μ + Bi + Gj + εij
with Yij = observation of genotype j in bloc i, μ = general mean, Bi = effect of bloc i , Gj = effect of genotype j, and εij = experimental error.
Then, lines were separated for GY using the least significant difference (LSD) test at a probability level of 5% to generate superior genotypes per environment.
Subsequently, combined ANOVA was applied to GY data using REML of PROC Mixed SAS (SAS Institute, 1988) to determine if G x E interaction effect was significant according to the following model:
Yijk = μ + Ei + Rj(Ei) + Gk + EiGk + εijk
with Yijk = observation of genotype k in environment i in replication j, μ = general mean, Ei = effect of environment i, Rj(Ei) = effect of replication j in environment i, Gk = effect of genotype k, EiGk = effect of genotype k x environment i interaction and εijk = experimental error.
PROC Mixed with the option of PDMix800 of SAS (SAS Institute, 1988) was implemented to compare means of barley genotypes across environments.
The AMMI model was performed using the GenStat (12.1) software to evaluate the significance and magnitude of G x E effect on grain yield according to the following model:
Yki = μ + αk + βi + Σnλnγgnδen + ρki
with Yki = observation of genotype k in environment i, μ = general mean, αk= genotype deviation, βi = environment deviation, λn= singular value for component n, γkn = eigenvector value for genotype k, δin = eigenvector value for environment i and ρki = experimental error.
-
Results and discussion
-
Grain yield performance per environment and across environments
During the growing season 2009-2010, the total rainfall was about 362 and 391 mm for Mograne and Boussalem respectively. However, temperatures ranged between 6 and 30°C for both sites. The rainfall during the cropping season (2010-2011) was superior to the previous. Total rainfall reached 459 and 537 mm for Mograne and Boussalem respectively. Temperatures varied between 4 and 30°C for both sites.
A wide range of variations was found among the barley lines for grain yield in each environment (Table 1). Summary statistics of the phenotypic performance of barley population for grain yield assessed in Mograne and Boussalem during 2009-2010 and 2010-2011 are represented in Table 2.
In Mograne during 2009-2010, grain yield ranged from 38.30 to 713.05 g/m2. The LSD (0.05) indicated that DH24, DH56, DH39, DH48, DH15, DH13, DH33, DH14, DH42 and DH53 were the most performing barley genotypes. The two barley lines DH24 and DH56 outyielded parental lines ‘Roho’ and ‘L90’. During the same growing season in Boussalem, grain yield varied from 27.46 to 800.35 g/m2 and best performances were obtained for genotypes DH38, DH20, DH24, DH58, DH48, DH44, DH14, DH60, DH28 and DH22. The majority of these barley lines performed better than parental lines. During the next season in Mograne, the lowest grain yield was about 32.18 g/m2 while the highest one was around 1242 g/m2. Lines DH24, DH38, DH28, DH19, DH44, DH48, DH49, DH62, DH14 and DH60 were the highest yielding barley genotypes. All these genotypes outperformed parents ‘Roho’ and ‘L90’. Barley grain yield ranged from 59.51 to 1203 g/m2 in Boussalem during 2010-2011. The LSD (0.05) indicated that DH58, DH38, DH24, DH28, DH44, DH48, DH59, DH50, DH46 and DH14 were the most performing barley genotypes but none of the genotype had exceeded the parent ‘Roho’. It can be concluded that environment Mg 2010-2011 was the best one for grain yield. Total rainfall during the vegetative growth was among the factors of barley grain yield expression in that environment. For the combined environments, DH24, DH38, DH48, DH44, DH14, DH28, DH49, DH58, DH62 and DH46 were the first ten yielding barley genotypes. Compared to the three barley checks Martin, Manel and Rihane, barley genotypes identified in each environment and in combined environments appeared more yielding.
Table 1. Analysis of variance per environment for grain yield in doubled-haploid barley lines |
|||||||||||
|
|
Environments |
|
|
|||||||
Source of variation |
Mg 2009-2010 |
BS 2009-2010 |
Mg 2010-2011 |
BS 2010-2011 |
|||||||
df |
MS |
MS |
MS |
|
MS |
||||||
Bloc |
2 395632.61ns |
264970.54ns |
866626.26ns |
38299.40ns |
|||||||
Genotype |
58 26324.23** |
18523.45** |
81447.88** |
59133.74** |
|||||||
Error |
116 8625.91 |
5328.72 |
18798.60 |
22828.93 |
|||||||
CV(%) |
32.33 |
28.50 |
26.25 |
31.96 |
|||||||
Mg: Mograne, BS: Boussalem, df: degree of freedom, MS: mean squares, **, ns: Significant at probability level of 1% and not significant at probability level of 5%. |
Table 2. The most performing doubled-haploid (DH) barley lines for grain yield evaluated across four environments |
|||||
Genotype rank |
Environments |
Combined environments |
|||
Mg 2009-2010 |
BS 2009-2010 |
Mg 2010-2011 |
BS 2010-2011 |
||
Genotype estimate |
Genotype estimate |
Genotype estimate |
Genotype estimate |
Genotype estimate |
|
1 |
DH24a (565.71) |
DH38a (459.32) |
DH24a (832.39) |
DH58a (770.73) |
DH24a (634.04) |
2 |
DH56a (540.77) |
DH20a (445.07) |
DH38a (824.43) |
DH38a (752.39) |
DH38b (596.98) |
3 |
DH39a (440.74) |
DH24a (394.07) |
DH28a (813.97) |
DH24a (744.01) |
DH48b (563.23) |
4 |
DH48b (411.19) |
DH58a (370.42) |
DH19a (802.17) |
DH28a (742.35) |
DH44c (538.06) |
5 |
DH15b (409.05) |
DH48a (366.46) |
DH44a (793.31) |
DH44a (706.14) |
DH14c (520.61) |
6 |
DH13b (408.14) |
DH44a (365.07) |
DH48a (791.56) |
DH48a (683.73) |
DH28c (519.45) |
7 |
DH33b (394.92) |
DH14a (361.27) |
DH49a (770.38) |
DH59a (673.70) |
DH49d (484.44) |
8 |
DH14b (372.02) |
DH60b (341.09) |
DH62a (743.54) |
DH50a (650.58) |
DH58d (474.83) |
9 |
DH42b (365.73) |
DH28b (337.24) |
DH14a (722.40) |
DH46a (642.57) |
DH62d (464.71) |
10 |
DH53c (356.06) |
DH22b (329.38) |
DH60a (709.73) |
DH14a (626.75) |
DH46d (457.36) |
‘L90’ |
481.48a |
304.75b |
705.14a |
523.11b |
503.76c |
‘Roho’ |
386.49b |
329.80b |
709.17a |
802.22a |
556.92a |
Martin |
281.17c |
192.46c |
444.72b |
394.99b |
328.33f |
Manel |
246.18c |
309.23b |
351.44c |
479.71b |
346.64f |
Rihane |
198.24d |
305.78b |
571.77b |
478.96b |
388.69e |
Min |
38.30 |
27.46 |
32.18 |
59.51 |
237.48 |
Mean |
287.23 |
256.05 |
522.14 |
472.65 |
383.07 |
Max |
713.05 |
800.35 |
1242 |
1203 |
634.04 |
LSD0,05 |
150.2 |
118.05 |
221.73 |
244.34 |
34.58 |
Mg: Mograne, BS: Boussalem, Values within the same column followed by the same letters are not significantly different according to LSD test (0.05). |
-
Assessment of G×E interaction for grain yield
The fact that each site identifies a specific set of performing barley genotypes is a powerful
indication of the presence of the G × E interaction. Table 2 showed that the rank of genotypes changes across the four environments. The combined analysis of variance demonstrated a highly significant difference for the environment effect (E), the genotype main effect (G) and genotype × environment interaction effect (G × E) (Table 3). Environmental effect was the most prominent contributing to the source of variation for 31.44% of the treatment sum of squares, while the genotype and G x E interaction effects explained 19.03% and 17.23% of the total variation, respectively. The large sum of squares for the environment effect indicated that environments were diverse causing variation in plant grain yields. This result is similar to that reported by Kadi et al. (2010) and Samonte et al. (2005) noting that the largest proportion of total variation in multi-environment trials is attributed to environments, whereas genotype and G × E interaction sources of variation are relatively smaller. Significant G × E interaction effects indicated that substantial differences in genotypic response across environments existed, suggesting to conduct multiple-environment trials to identify superior cultivars for the target region. Previous studies have also reported significant G × E interaction effect for grain yield in barley populations (Von Korff et al. 2008; Schmalenbach et al. 2009).
Table 3. Combined analysis of variance for grain yield in doubled-haploid barley lines evaluated across four environments |
|||
Source of variation |
df |
MS |
% of variation explained |
Environment |
3 |
3107868.60** |
31.44 |
Repetition (Environment) |
8 |
391382.20 |
10.56 |
Genotype |
58 |
97336.59** |
19.03 |
Genotype x Environment |
174 |
29364.24** |
17.23 |
Error |
464 |
13895.54 |
21.74 |
CV (%) |
|
30.65 |
|
MS: mean squares, df: degree of freedom, **: Significant at probability level of 1%. |
The G x E interaction for barley grain yield, as outlined in the ANOVA, was explained by the AMMI model. Actually, the first two terms of AMMI analysis were significant, explaining 84.47% of the G × E interaction sum of squares (52.71% and 31.76% for the first and the second interaction principal component axis, respectively) (Table 4). The AMMI model appeared effective in capturing a large portion of the G × E sum of squares. Li et al. (2006) demonstrated the effectiveness of the AMMI model to investigate G × E interaction effects in Chinese spring wheat. Its efficiency has also been proven in Mediterranean environments for G × E interaction effects on yield and yield components in barley genotypes (Van Oosterom et al. 1993; Voltas et al. 1999).
Table 4. The Additive Main effect and Multiplicative Interaction analysis of variance for grain yield in doubled-haploid barley lines evaluated across four environments |
|||
Source of variation |
df |
MS |
% of variation explained |
Treatments |
235 |
85441** |
|
Genotypes |
58 |
97337** |
|
Environments |
3 |
3107868** |
|
Block |
8 |
391382 |
|
Genotypes × Environment |
174 |
29364** |
|
IPCA1 |
60 |
44891** |
52.71 |
IPCA2 |
58 |
27986** |
31.76 |
Residuals |
56 |
14155 ns |
|
Error |
464 |
13896 |
|
Total |
707 |
41948 |
|
MS: mean squares, df: degree of freedom, **, ns: Significant at probability level of 1% and not significant at probability level of 5%. |
This G x E interaction for grain yield indicated that DH24, DH48 and DH14 were four times among the ten most yielding barley genotypes (Table 2). Genotypes DH38 and DH28 performed best three times. However, DH44 and DH58 were twice among the top ten genotypes. Thus, these genotypes could be considered as stable barley genotypes for grain yield.
-
Conclusion
The results indicated that there were desirable genotypes in the barley population in terms of high mean yields over the four tested environments. Lines DH24, DH38 and DH48 were the most productive genotypes. Grain yield performance was highly influenced by G × E interaction effects. The presence of the genotype x environment interaction indicated a change of genotypes rank across environments. The AMMI model effectively explained 84.72% of G × E interaction. Moreover, results identified DH24, DH48, DH14, DH38, DH28, DH44 and DH58 as the seven best performing and stable barley lines. Therefore, it is reasonable to recommend these varieties as progenitors in barley breeding programs for high grain yield.
Acknowledgments
We thank Dr. Pierre Devaux and his team from seed Coop. ‘Florimond Desprez Veuve and Fils’ for their contribution in this investigation by producing doubled haploid lines.
-
References
Choo TM, Reinbergs E and Kasha KJ (1985) Use of haploids in breeding barley. Plant Breeding 3: 219-252.
El-Soda M, Malosetti M, Zwaan BJ, Koornneef M, and Aarts MGM (2014) Genotype x environment interaction QTL mapping in plants: lessons from Arabidopsis. Trends in Plant Science: 1-9.
Food Agricultural Organisation (FAO) (2013) FAOSTAT< URL: www.faostat.FAO.org>.
Kadi Z, Ajel F, Bouzerzour H (2010) Analysis of the Genotype X Environment Interaction of Barley Grain Yield (Hordeum Vulgare L.) under Semi Arid Conditions. Advance in Environmental Biology 4: 34-40.
Li W, Yan ZH, Wei YM, Lan XJ and Zheng YL (2006) Evaluation of Genotype x environment interactions in Chinese spring wheat by the AMMI model, correlation and path analysis. Crop Sciences 192: 221–227.
Malosetti M, Ribaut JM and vanEeuwijk FA (2013) The statistical analysis of multi-environment data: modeling genotype-by-environment interaction and its genetic basis. Frontiers in Physiology (4) 44: 1-17.
Pickering R and Devaux P (2005) Haploids in the improvement of Graminaceous species, Haploids in crop improvement. Springer Heidelberg: 214-242.
Romagosa I and Fox PN (1993) Genotype by environment interaction and adaptation. IMD Hayward, NO Bosemark, In Romagosa. eds, Plant Breeding: Principles and Prospects, Chapman & Hall, London, pp 373-390.
Samonte SO, Wilson LT, McClung AM and Medley JC (2005) Targeting cultivars onto rice growing environments using AMMI and SREG GGE biplot analyses. Crop Sciences 45: 2414-2424.
SAS Institute (1988) SAS/ETS User’s Guide - Version 6 Edition. SAS Institute Inc., Box 8000, Cary, N.C. 27512.
Schmalenbach I, Léon J, Pillen K (2009) Identification and verification of QTLs for agronomic traits using wild barley introgression lines. Theoretical and Applied Genetics 118: 483-497.
Van Oosterom EJ, Kleijn D, Ceccarelli S and Nachit MM (1993) Genotype x environment interactions of barley in the Mediterranean region. Crop Sciences 33: 669-674.
Voltas J, Romagosa I, Lafarga A, Armesto AP, Sombrero A and Araus JL (1999) Genotype by environment interaction for grain yield and carbon isotope discrimination of barley in Mediterranean Spain. Australian Journal of Agricultural Researches 50: 1263-1271.
Von Korff M, Grando S, Del Greco A, This D, Baum M, Ceccarelli S (2008) Quantitative trait loci associated with adaptation to Mediterranean dryland conditions in barley. Theoretical and Applied Genetics 117: 653-669.
Yan W, Hunt LA, Sheng Q and Szlavnics Z (2000) Cultivar evaluation and mega-environment investigation based on the GGE biplot. Crop Sciences 40: 597-605.