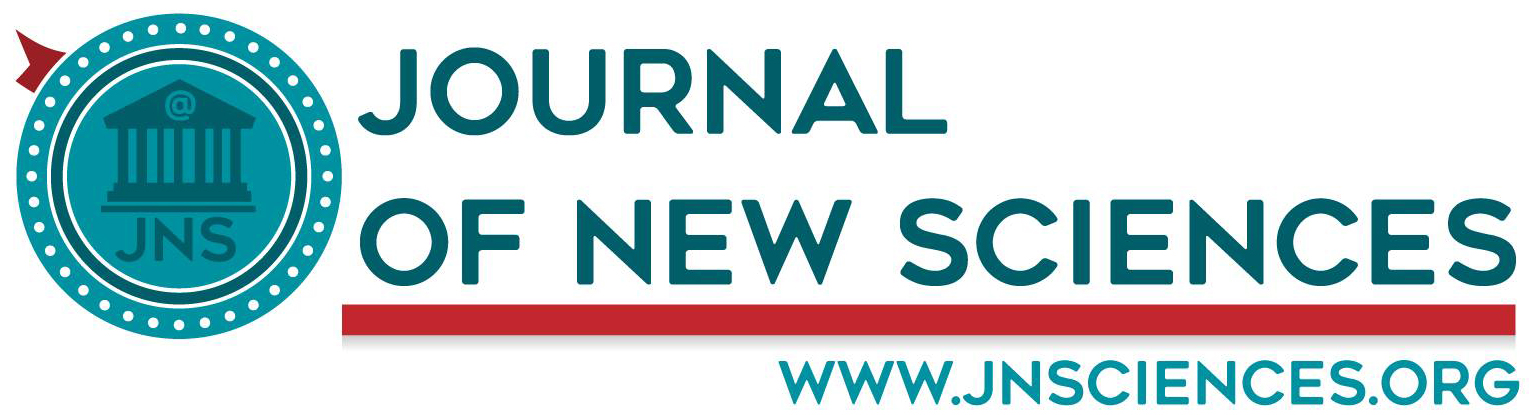
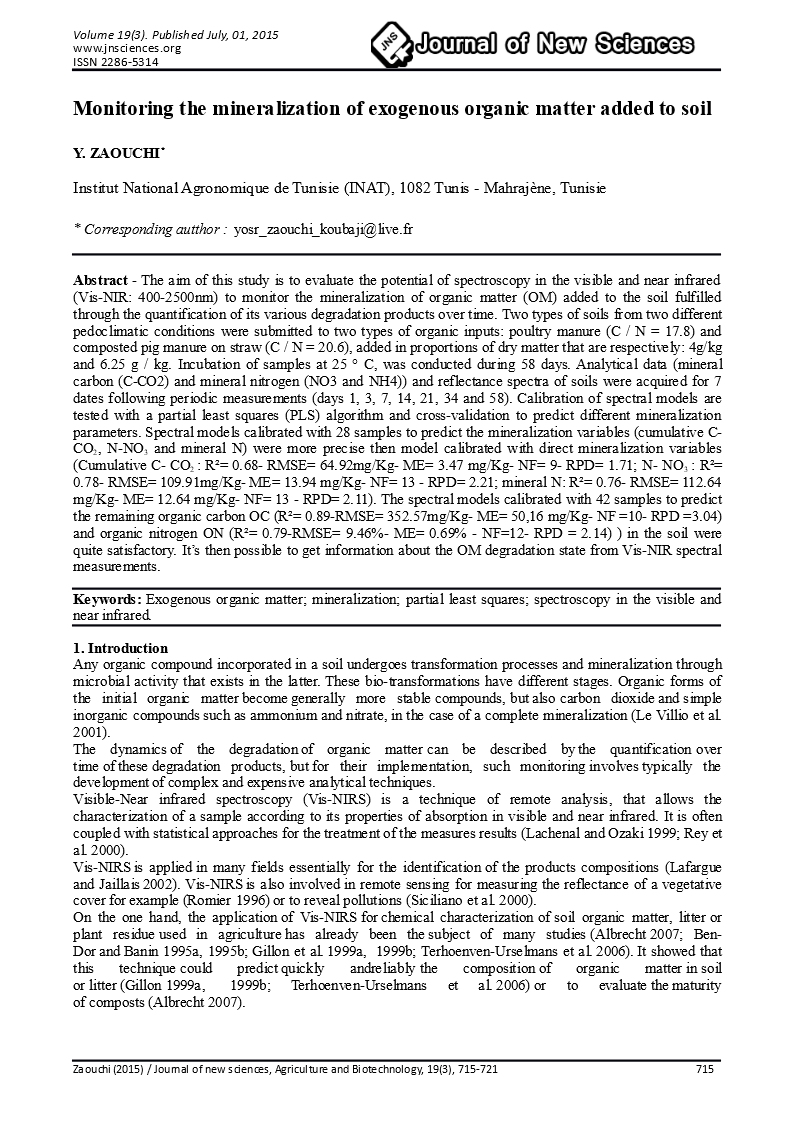
- Category: Volume 19
- Hits: 5461
Monitoring the mineralization of exogenous organic matter added to soil
Y. Zaouchi *
Institut National Agronomique de Tunisie (INAT), 1082 Tunis - Mahrajène, Tunisie
Abstract - The aim of this study is to evaluate the potential of spectroscopy in the visible and near infrared (Vis-NIR: 400-2500nm) to monitor the mineralization of organic matter (OM) added to the soil fulfilled through the quantification of its various degradation products over time. Two types of soils from two different pedoclimatic conditions were submitted to two types of organic inputs: poultry manure (C / N = 17.8) and composted pig manure on straw (C / N = 20.6), added in proportions of dry matter that are respectively: 4g/kg and 6.25 g / kg. Incubation of samples at 25 ° C, was conducted during 58 days. Analytical data (mineral carbon (C-CO2) and mineral nitrogen (NO3 and NH4)) and reflectance spectra of soils were acquired for 7 dates following periodic measurements (days 1, 3, 7, 14, 21, 34 and 58). Calibration of spectral models are tested with a partial least squares (PLS) algorithm and cross-validation to predict different mineralization parameters. Spectral models calibrated with 28 samples to predict the mineralization variables (cumulative C-CO2, N-NO3 and mineral N) were more precise then model calibrated with direct mineralization variables (Cumulative C- CO2 : R²= 0.68- RMSE= 64.92mg/Kg- ME= 3.47 mg/Kg- NF= 9- RPD= 1.71; N- NO3 : R²= 0.78- RMSE= 109.91mg/Kg- ME= 13.94 mg/Kg- NF= 13 - RPD= 2.21; mineral N: R²= 0.76- RMSE= 112.64 mg/Kg- ME= 12.64 mg/Kg- NF= 13 - RPD= 2.11). The spectral models calibrated with 42 samples to predict the remaining organic carbon OC (R²= 0.89-RMSE= 352.57mg/Kg- ME= 50,16 mg/Kg- NF =10- RPD =3.04) and organic nitrogen ON (R²= 0.79-RMSE= 9.46%- ME= 0.69% - NF=12- RPD = 2.14) ) in the soil were quite satisfactory. It’s then possible to get information about the OM degradation state from Vis-NIR spectral measurements.
Keywords: Exogenous organic matter; mineralization; partial least squares; spectroscopy in the visible and near infrared.
1. Introduction
Any organic compound incorporated in a soil undergoes transformation processes and mineralization through microbial activity that exists in the latter. These bio-transformations have different stages. Organic forms of the initial organic matter become generally more stable compounds, but also carbon dioxide and simple inorganic compounds such as ammonium and nitrate, in the case of a complete mineralization (Le Villio et al. 2001).
The dynamics of the degradation of organic matter can be described by the quantification over time of these degradation products, but for their implementation, such monitoring involves typically the development of complex and expensive analytical techniques.
Visible-Near infrared spectroscopy (Vis-NIRS) is a technique of remote analysis, that allows the characterization of a sample according to its properties of absorption in visible and near infrared. It is often coupled with statistical approaches for the treatment of the measures results (Lachenal and Ozaki 1999; Rey et al. 2000).
Vis-NIRS is applied in many fields essentially for the identification of the products compositions (Lafargue and Jaillais 2002). Vis-NIRS is also involved in remote sensing for measuring the reflectance of a vegetative cover for example (Romier 1996) or to reveal pollutions (Siciliano et al. 2000).
On the one hand, the application of Vis-NIRS for chemical characterization of soil organic matter, litter or plant residue used in agriculture has already been the subject of many studies (Albrecht 2007; Ben-Dor and Banin 1995a, 1995b; Gillon et al. 1999a, 1999b; Terhoenven-Urselmans et al. 2006). It showed that this technique could predict quickly andreliably the composition of organic matter in soil or litter (Gillon 1999a, 1999b; Terhoenven-Urselmans et al. 2006) or to evaluate the maturity of composts (Albrecht 2007).
On the other hand, no research has focused on the ability of Vis-NIRS in monitoring the temporal evolution of an organic matter brought to a soil. However, the Vis-NIRS technique could be faster, cheaper and more accessible than traditional techniques used for monitoring the dynamics of mineralization of the exogenous MO. If the state of degradation of organic matter applied to the soil was affected by the reflectance in the range of wavelengths in the near infrared, it would be possible to follow the mineralization of organic matter by spectroscopy with a temporal resolution which is not accessible to conventional analytical techniques.
The overall objective of this study is to evaluate the potential of Spectrometry in the visible-near infrared to monitor the mineralization of the EOM made to a soil, by quantifying over time, its various degradation products.
2. Materials and methods
In this study, two soils of different pedo-climatic origins have received two kinds of organic inputs. Monitoring the mineralization of both EOM is performed through periodic mineral analyzes. These data were then compared with the change in time of reflectance spectra of soil samples with statistical analysis. Then, test calibrations of spectral models were produced for the predictions of various mineralization parameters.
The experiment was conducted at the mixed Research Unit - Soil Agro and hydrosystem Spatialisation (Unité mixte de Recherche Sol Agro et hydrosystème Spatialisation(UMR SAS)) of the Agrocampus Ouest Located in Rennes- France.
2.1. Experimental material
The experiment focused on surface horizons of two cultivated soils occurring in different pedoclimatic conditions. A soil (Vertic Fluvisols) from the region of Mornag situated in the northeast of Tunisia and more exactly in the southeast of Tunis. This soil is composed of 0.75% of organic C , 37 % of clay and 42 % of silt. The other soil (Eutric Luvisol developed on Aeolian loam) results from an experimental plot of land, "Champ Noël", situated in the North of Rennes (Brittany, France) and it is a part of an experimental domain of the INRA. This soil is composed of 1.05% of organic C, 14% of clay and 49% of silt. The C/N report of the French soil is 5.8 while that of Tunisia is 7.06. The latter presents a relatively important percentage of carbonates, corresponding to 24.7 % of the mass of the dry soil.
Two types of amendments with different biodegradability are mixed with samples of both soil types, poultry manure (C / N = 17.8) from a commercial breeding of Morbihan, and pig manure composted straw (C / N = 20.6) from an experimental station of the Agriculture Chamber of Guernévez (Finistère, France). The dose of contribution of these two EOM is calculated so that the soil is added to the same quantities of carbon and nitrogen.
Incubation in the dark in a controlled environment room, (temperature set at 25 ° C) was performed for soil samples for 58 days.
2.2. Sample preparation
Soil samples were collected on a layer of 0 -20 cm and were sieved to less than 2 mm and stored in the fridge for one month before the start of the experiment. Soil moisture was then adjusted to field capacity. The various samples were then left to stand for 4 days before being incubated to allow the recovery of biological soil activity in order to avoid the "flush" effect (Bernard 1981).
For each treatment, three repetitions are performed for each treatment. Soil samples are conditioned in a series of glass jars hermetically closed. A pot of ultra pure water is put inside to keep the atmosphere saturated with moisture and prevent rapid drying of samples. A pot of 1M sodium hydroxide and volume equal to 20ml is also placed inside the jar in order to trap the CO2 produced by microbial respiration. A jar which only contains pots of sodium hydroxide and water, serves as a blank to measure CO2 in its atmosphere.
2.3. Periodic measurements
Periodic sampling is performed at different times: days 1, 3, 7, 14, 21, 34 and 58 of the incubation period. In fact, at each date, CO2 and mineral nitrogen (NO3 and NH4) produced by the various samples are measured. The determination of the CO2 is made by titration with the hydrochloric acid. At each sampling date, pots of sodium hydroxide are replaced for the next date. The quantity of carbon mineralized by microorganisms is calculated in mg / kg dry soil, via the volume of the hydrochloric acid used to neutralize one millilitre of sodium hydroxide, which allows to know the quantity of unreacted sodium hydroxide, that combined with the CO2 via substraction. The mineral nitrogen is extracted by a solution of potassium chloride KCl. The ammonium and nitrate ions are determined by continuous flow colorimetry using the reaction of Griess-Ilosvay for nitrates. The absorbance is measured in 550nm. The ammonium is measured using the reaction of Berthelot, in that case the absorbance is measured in 660nm (Duclaux 1965).
2.4. Acquisition of spectral data
The acquisition of the spectral data of the various samples of soils were achieved the same day as the conditioning (mineral nitrogen, CO2) so that the spectral measures and those of laboratory are made for the same stage of mineralization of the organic matter. The recording of spectres is made by a visible-near infrared spectrometer ASD FieldSpec® (Analytical Spectral Devices, Inc., Boulder, CO, USA) operating in a range of wavelengths ranging from 350nm to 2500nm with a step of 1nm. For each sample, 4 measures are made and for each of these measures, 10 spectres are acquired. Only their average value is taken into account. These 4 measures are obtained by turning 4 times the capsule that contains the soil sample with a 90 ° angle, to eliminate the directional effect caused by the heterogeneousness of the surface (Karnieli et al. 1999), but also to compensate for any defects of symmetry of the lighting device.
2.5. Calibration tests of a model to predict the dynamics of OM mineralization using analytical data and spectra through the method of partial least squares (PLS)
In this part of data analysis, the objective is to determine parameters describing the mineralization of the organic matter in various treatments which are best predicted from the spectral data. The model is adjusted by cross-validation according to the "leave one out» method. The chosen model presents the highest R ² and the lowest RMSE and ME.
3. Results
Figure 1 represent the mean spectrum for each treatment modality. Spectra significantly differ according to the nature of the EOM and the type of soil.
|
Figure 1: mean spectrum for each treatment modality all dates combined. |
Firstly, PLS calibrated models with direct analytical values of carbon, inorganic nitrogen, ammonia and nitric acid, have been unsatisfactory (C-CO2: R ² = 1 - RMSE = 183.3 mg / kg - ME = -0.1 mg / kg; mineral-N: R ² = 0.29 - RMSE = 257.4 mg / kg - ME = 4.9 mg / kg; N-NH4 R ² = 0.01 - RMSE = 41.7 mg / kg - ME = 0.5 mg / kg; NO3-N: R ² = 0.2 - RMSE = 284mg/kg - ME = 15.2 mg / kg) .
After this observation, it has been decided to test other parameters derived from those used for previous calibrations, describing the dynamics of mineralization of the EOM as calculated cumulative carbon resulting from the mineralization of the EOM, and total nitric and ammoniacal nitrogen, also resulting from the mineralization of the EOM (figure 2).
For these parameters, the data resulting from subtraction of the values of the control soils without amendment from soils containing EOM. These new calibrations were performed with 28 samples of soil with EOM. Thus, they have improved performance prediction models of mineralization parameters such as the cumulative quantity of carbon mineralized, inorganic nitrogen and nitrate nitrogen, both resulting from the mineralization of the EOM (Cumulative C- CO2 : R²= 0.68- RMSE= 64.92mg/Kg- ME= 3.47 mg/Kg- NF= 9- RPD= 1.71; N- NO3 : R²= 0.78- RMSE= 109.91mg/Kg- ME= 13.94 mg/Kg- NF= 13 - RPD= 2.21; mineral N: R²= 0.76- RMSE= 112.64 mg/Kg- ME= 12.64 mg/Kg- NF= 13 - RPD= 2.11) (Figure 2).
To make these models more robust, much larger data set should be used. The coefficient of determination (R²) must be greater than or equal to 0.82, in order to consider the model as accurate (De Girolamo et al. 2009).
Total nitrogen from EOM (mg/kg soil) |
Figure 2: Comparison of predicted and observed values for a PLS model quantities (mg / Kg of soil) mineralized carbon (a), total nitrogen (b) and nitric nitrogen (c), all resulting from the EOM mineralization. |
Other calibrations were carried out from the values of organic carbon and nitrogen remaining in the soil. For the implementation of these models, 42 samples were used including soils without inputs and those containing the EOM. The resulting models for the prediction of these two parameters are more precise than those for not only carbon and mineral nitrogen but also for mineralized ammoniacal and nitric nitrogen (Figure 3). In fact, the spectral models calibrated to predict the remaining organic carbon OC (R²= 0.89-RMSE= 352.57mg/Kg- ME= 50,16 mg/Kg- NF =10- RPD =3.04) and organic nitrogen ON (R²= 0.79-RMSE= 9.46%- ME= 0.69% - NF=12- RPD = 2.14) ) in the soil were quite satisfactory.
R²=0.89 - RMSE=352.57mg/kg ME=-50.16mg/kg – NF=10 RPD=3.04
R²=0.79 – RMSE=9.46% ME=-0.69% – NF=12 RPD=2.14
(a) (b) R²=0.89 RMSE=352.57mg/kg ME=-50.16mg/kg NF=10 RPD=3.04
R²=0.89 RMSE=352.57mg/kg ME=-50.16mg/kg NF=10 RPD=3.04
|
Figure 3: Comparison of predicted and observed values of a PLS model of carbon (a) (mg / Kg of soil) and organic nitrogen; (b) (%) remaining in the soil. |
4. Discussion
Spectral models calibrated with 28 samples to predict the mineralization variables (cumulative C-CO2, N-NO3 and mineral N) were more precise then model calibrated with direct mineralization variables (Cumulative C- CO2, N- NO3 and mineral N). The spectral models calibrated with 42 samples to predict the remaining organic carbon OC and organic nitrogen ON in the soil were quite satisfactory.
The prediction of soil carbon by Vis-NIR has been successful in other works (Chang and Laird 2002; Ludwig et al. 2002; Schimann et al. 2007; Bellon-Maurel and McBratney 2011). The potential of Vis-NIRS to predict total nitrogen has been reported in several works, such as arable, forest and mineral soils and grasslands (Chodak 2008; Schimann et al. 2007). Chang and Laird (2002) and Terhoeven-Urselmans et al. (2006, 2008) were able to obtain reliable models to predict C / N ratio with Vis-NIRS. Contradictory results were obtained by Ludwig et al. (2002). St. Luce et al. (2014) used the Vis-NIR (400-2498 nm) to predict the evolution of total nitrogen, organic carbon and C / N ratios of Chernozemic soils of western Canada. They tested 146 samples for the calibration of modified PLS models and 49 others for validation. The Vis-NIRS predictions were considered reliable for total nitrogen and soil organic carbon (R2V > 0.80, RPDV > 2.4), but they are less for C / N ratio (R2V = 0.54, RPDV = 1.45). Other authors have confirmed the reliability of the Vis NIRS for the prediction of the total nitrogen and soil organic carbon (Chang and Laird 2002; Shepherd et Walsh, 2002 ; Brunet et al, 2007; Zornoza et al, 2008 ; Nduwamungu et al, 2009 ; Xie et al. 2011 ; St. Luce et al. 2012; Shi et al. 2013). However, Vohland et al. (2014) obtained accurate results for the organic carbon (CO) and nitrogen (N) predictions using the mid-infrared spectrum rather than the Vis-NIR. According to Fystro (2002), the quality of the obtained models for the prediction of total nitrogen and organic carbon of the soil is related to the high correlation (r = 0.98) between these two parameters. According to St. Luce et al. (2014), the positive correlations are observed in the NIR region (2148nm for soil total nitrogen, 2156nm for soil organic carbon and 1400nm for C/N ratio). Negative correlations are observed in the visible region at 546 nm for total nitrogen and organic carbon of the soil but they are at 1426nm for the C/N ratio.
Our primary results are satisfactory. However, the models obtained could still be improved. To increase the chances of success of these calibrations, it would be better to increase the number of repetitions.
Actually, instead of a global calibration by simultaneously integrating the two parameters (soil parameters and exogenous organic matter), it would be better to test the two soils with the same input, then the same ground with the two inputs. The use of two soils and two effluents of different natures and origins could have made the tested samples too heterogeneous, thus decreasing the accuracy of different calibrated models. According to Shi et al. (2013) and Xie et al. (2011), the use of soil samples taken from the same field or the same type of soil would produce accurate models. One hypothesis to explain the weakness of a predictive model is the ability of the Vis-NIRS to detect a wide combination of soil properties that contain various chemical bonds, such as clay content (St. Luce et al. 2014).
5. Conclusion
Calibrated models spectra to predict some parameters describing the dynamics of mineralization of EOM, such as cumulative carbon resulting from the mineralization of EOM, or total nitrogen and nitrate also resulting from the same process, are quite satisfactory. However, the spectral models for predicting some parameters which can indicate indirectly the state of mineralization of EOM, such as organic carbon and organic nitrogen remaining in the soil, have been even more accurate. These initial results show that the visible near-infrared spectroscopy could be a reliable tool for monitoring changes in organic inputs and their mineralization in soil.
6. References
Albrecht R (2007). thesis: Co-compostage de boues de station d’épuration et de déchets verts : nouvelle méthodologie du suivi des transformations de la matière organique. Université Paul Cézanne Aix-Marseille III. France.
Bellon-Maurel V, McBratney A (2011). Near-infrared (NIR) and mid-infrared (MIR) spectroscopic techniques for assessing the amount of carbon stock in soils – critical review and research perspectives. Soil Biol. Biochem. 43: 1398–1410.
Ben-Dor E, Banin A (1995a). Near-infrared analyses as a rapid method to simultaneously evaluate several soil properties. Soil Science Society of America Journal. 59: 364-372.
Ben-Dor E, Banin A (1995b). Near-infrared analyses as a rapid method to simultaneously evaluate spectral featureless constituents in soil. Soil Science. 159: 259-270.
Bernard F (1981). Note sur l’influence du régime thermique et hydrique sur l’ammonification et la nitrification dans un sol de savane sahélienne. Cahier ORSTOM. 18 (2): 147-152.
Brunet D, Barthès BG, Chotte JL, Feller C (2007). Determination of carbon and nitrogen contents in Alfisols, Oxisols and Ultisols from Africa and Brazil using NIRS analysis: effects of sample grinding and set heterogeneity. Geoderma. 139: 106–117.
Chang CW, Laird DA (2002). Near-infrared reflectance spectroscopic analysis of soil C and N. Soil Sci. 167: 110–116.
Chodak M (2008). Application of near infrared spectroscopy for analysis of soils, litter and plant material. A review. Pol. J. Environ. Stud. 17: 631–642.
De Girolamo A, Lippolis V, Nordkvist E, Visconti A (2009). Rapid and non-invasive analysis of deoxynivalenol in durum and common wheat by Fourier-Transform Near Infrared (FT-NIR) spectroscopy. Food Additives and Contaminants A. 26: 907-917
Duclaux E (1965). Annales de l’Institut Pasteur. Institut Pasteur, CNRS. 109 (2) : 517. Masson, Paris.
Fystro G (2002). The prediction of C and N content and their potential mineralisation in heterogeneous soil samples using Vis-NIR spectroscopy and comparative methods. Plant Soil. 246: 139–149.
Gillon D, Houssard C, Joffre R (1999a). Using near-infrared reflectance spectroscopy to predict carbon, nitrogen and phosphorus content in heterogeneous plant material. Oecologia. 118: 173-182.
Gillon D, Joffre R, Ibrahima A (1999b). Can litter decomposability be predicted by near-infrared reflectance spectroscopy? Ecology. 80: 175-186.
Karnieli A, Kidron GJ, Glaesser C, Ben-Dor E (1999). Spectral Characteristics of Cyanobacteria Soil Crust in Semiarid Environments. Remote Sensing of Environment. 69: 67-75.
Lachenal G, Ozaki Y (1999). Advantages of near infrared spectroscopy for the analysis of polymers and composites. Macromolecular symposia. 141 : 283.
Lafargue M, Jaillais B (2002). La spectroscopie proche infrarouge dans l'agroalimentaire : Principes et instrumentation, traitement des données, applications. Spectra 2000 analyse. 31 : 15-22
Le Villio M, Arrouays D, Deslais W, Daroussin J, Le Bissonnais Y, Clergeot D (2001). Estimation des quantités de matière organique exogène nécessaires pour restaurer et entretenir les sols limoneux français à un niveau organique donné. Étude et Gestion des Sols. 94 : 47- 63.
Ludwig B, Khanna PK, Bauhus J, Hopmans P (2002). Near infrared spectroscopy of forest soils to determine chemical and biological properties related to soil sustainability. Forest Ecol. Manage. 171: 121–132.
Nduwamungu C, Ziadi N, Tremblay GF, Parent LÉ (2009b). Near-infrared reflectance spectroscopy prediction of soil properties: effects of sample cups and preparation. Soil Sci. Soc. Am. J. 73: 1896–1903.
Rey L, Galy J, Sautereau H, Lachenal G, Henry D, Vial J (2000). Near-Infrared Spectroscopy for in Situ Cure Monitoring of Dimethacrylate-Based Networks. Applied Spectroscopy. 54 : 39-43.
Romier J (1996). Thesis : Conception et réalisation d'un spectromètre imageur visible et proche infrarouge. Université de Toulouse 3, Toulouse, France (Université de soutenance).
Schimann H, Joffre R, Roggy JC, Lensi R, Domenach AM (2007). Evaluation of the recovery of microbial functions during soil restoration using near-infrared spectroscopy. Appl. Soil Ecol. 37: 223–232.
Shi T, Cui L, Wang J, Fei T, Chen Y, Wu G (2013). Comparison ofmultivariate methods for estimating soil total nitrogen with visible/near-infrared spectroscopy. Plant Soil. 366: 363–375.
Siciliano SD, Roy R, Greer CW (2000). Reduction in denitrification activity in field soils exposed to long term contamination by 2,4,6-trinitrotoluene (TNT). FEMS Microbiology Ecology. 32: 61-68.
Shepherd KD, Walsh MG (2002). Development of reflectance spectral libraries for characterization of soil properties. Soil Sci. Soc. Am. J. 66: 988–998.
St. Luce M, Ziadi N, Nyiraneza J, Tremblay GF, Zebarth BJ,Whalen JK, Laterrière M (2012). Near infrared reflectance spectroscopy prediction of soil nitrogen supply in humid temperate regions of Canada. Soil Sci. Soc. Am. J. 76: 1454–1461.
St. Luce M, Ziadi N, Zebarthb BJ, Grant CA, Tremblay GF, Gregorich EG (2014). Rapid determination of soil organic matter quality indicators using visible near infrared reflectance spectroscopy. Geoderma. 232 (234): 449–458.
Terhoenven-Urselmans T, Michel K, Helfrich M, Flessa H, Ludwig B (2006). Near infrared spectroscopy can predict the composition of organic matter in soil and litter. Journal of Plant Nutrition and Soil Science .169: 168-174.
Terhoeven-Urselmans T, Schmidt H, Joergensen RG, Ludwig B (2008). Usefulness of near-infrared spectroscopy to determine biological and chemical soil properties: importance of sample pre-treatment. Soil Biol. Biochem. 40: 1178–1188.
Vohland M, Ludwig M, Thiele-Bruhnb S, Ludwig B (2014). Determination of soil properties with visible to near- and mid-infrared spectroscopy: Effects of spectral variable selection. Geoderma. 223 (225) : 88–96.
Xie, HT, Yang XM, Drury CF, Yang JY, Zhang XD (2011). Predicting soil organic carbon and total nitrogen using mid- and near-infrared spectra for Brookston clay loam soil in Southwestern Ontario, Canada. Can. J. Soil Sci. 91: 53–63.
Zornoza R, Guerrero C, Mataix-Solera J, Scow KM, Arcenegui V, Mataix-Beneyto J, (2008). Near infrared spectroscopy for determination of various physical, chemical and biochemical properties in Mediterranean soils. Soil Biol. Biochem. 40: 1923–1930.