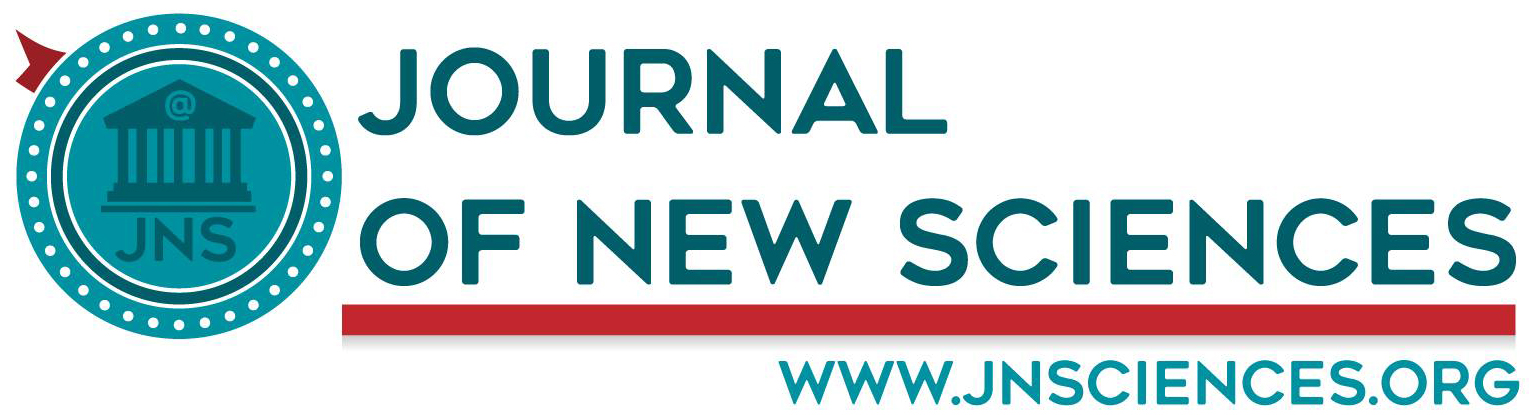
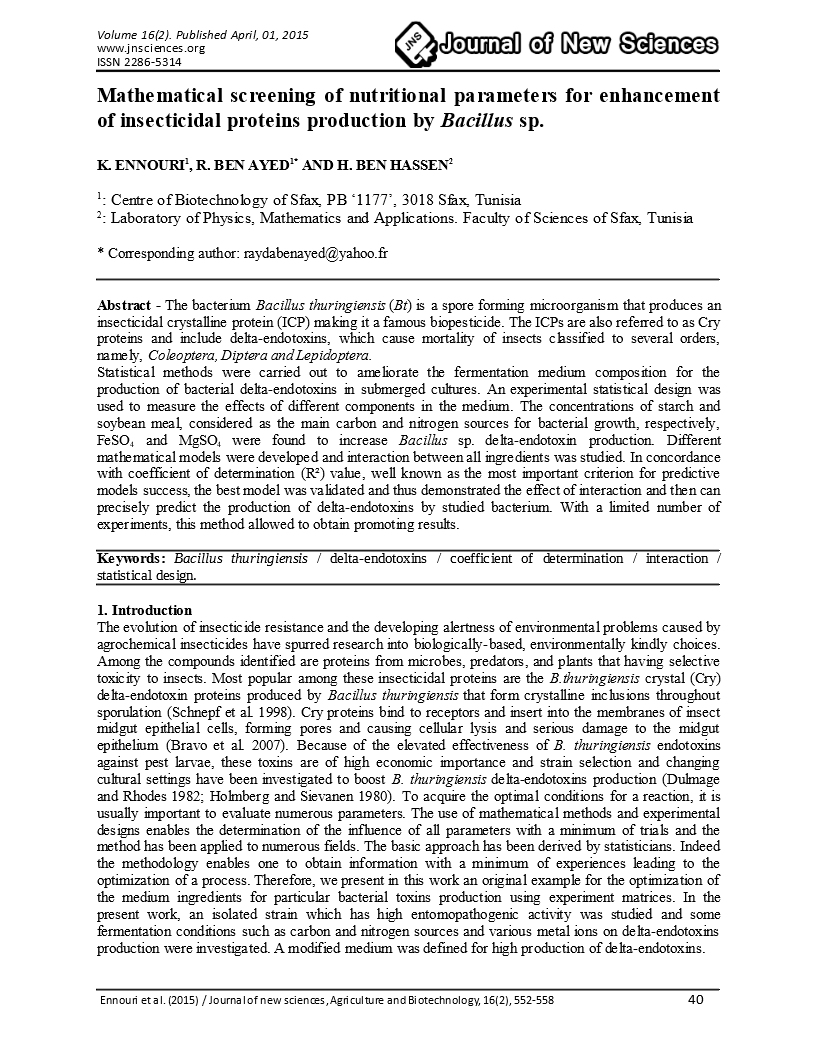
- Category: Volume 16
- Hits: 6724
Mathematical screening of nutritional parameters for enhancement of insecticidal proteins production by Bacillus sp.
K. Ennouri1
R. Ben Ayed1*
H. Ben Hassen2
1 Centre of Biotechnology of Sfax, PB ‘1177’, 3018 Sfax, Tunisia
2 Laboratory of Physics, Mathematics and Applications. Faculty of Sciences of Sfax, Tunisia
Abstract - The bacterium Bacillus thuringiensis (Bt) is a spore forming microorganism that produces an insecticidal crystalline protein (ICP) making it a famous biopesticide. The ICPs are also referred to as Cry proteins and include delta-endotoxins, which cause mortality of insects classified to several orders, namely, Coleoptera, Diptera and Lepidoptera.
Statistical methods were carried out to ameliorate the fermentation medium composition for the production of bacterial delta-endotoxins in submerged cultures. An experimental statistical design was used to measure the effects of different components in the medium. The concentrations of starch and soybean meal, considered as the main carbon and nitrogen sources for bacterial growth, respectively, FeSO4 and MgSO4 were found to increase Bacillus sp. delta-endotoxin production. Different mathematical models were developed and interaction between all ingredients was studied. In concordance with coefficient of determination (R²) value, well known as the most important criterion for predictive models success, the best model was validated and thus demonstrated the effect of interaction and then can precisely predict the production of delta-endotoxins by studied bacterium. With a limited number of experiments, this method allowed to obtain promoting results.
Keywords: Bacillus thuringiensis / delta-endotoxins / coefficient of determination / interaction / statistical design.
1. Introduction
The evolution of insecticide resistance and the developing alertness of environmental problems caused by agrochemical insecticides have spurred research into biologically-based, environmentally kindly choices. Among the compounds identified are proteins from microbes, predators, and plants that having selective toxicity to insects. Most popular among these insecticidal proteins are the B.thuringiensis crystal (Cry) delta-endotoxin proteins produced by Bacillus thuringiensis that form crystalline inclusions throughout sporulation (Schnepf et al. 1998). Cry proteins bind to receptors and insert into the membranes of insect midgut epithelial cells, forming pores and causing cellular lysis and serious damage to the midgut epithelium (Bravo et al. 2007). Because of the elevated effectiveness of B. thuringiensis endotoxins against pest larvae, these toxins are of high economic importance and strain selection and changing cultural settings have been investigated to boost B. thuringiensis delta-endotoxins production (Dulmage and Rhodes 1982; Holmberg and Sievanen 1980). To acquire the optimal conditions for a reaction, it is usually important to evaluate numerous parameters. The use of mathematical methods and experimental designs enables the determination of the influence of all parameters with a minimum of trials and the method has been applied to numerous fields. The basic approach has been derived by statisticians. Indeed the methodology enables one to obtain information with a minimum of experiences leading to the optimization of a process. Therefore, we present in this work an original example for the optimization of the medium ingredients for particular bacterial toxins production using experiment matrices. In the present work, an isolated strain which has high entomopathogenic activity was studied and some fermentation conditions such as carbon and nitrogen sources and various metal ions on delta-endotoxins production were investigated. A modified medium was defined for high production of delta-endotoxins.
2. Experimental Methods
2.1. The B. thuringiensis strain
The studied strain of B. thuringiensis subsp. kurstaki is provided from the collection of bacterial strains (Center of Biotechnology of Sfax, Tunisia), known for its toxicity against Lepidoptera insects (Ennouri et al. 2013). The bacterial strain was grown for maintained on Luria Bertani (LB) agar medium composed of 10 g l-1 peptone, 5g l-1 NaCl, 5g l-1 yeast extract and 15 g l-1 agar and then stored at 4 °C.
2.2. Microorganism and cultivation media
For fermentation medium, an economic complex medium (Ghribi et al. 2007) was used containing the following components (g l-1): starch, 25; soybean meal, 20; MgSO4, 0.3; MnSO4, 0.02; FeSO4, 0.02; K2HPO4, 1; KH2PO4, 1;. CaCO3 (20 g l-1) was added for keeping pH stability. All media used in this study were adjusted to pH 7.0 ± 0.01 before autoclaving.
2.3. Culture conditions
For pre-inoculum preparation, a loopful of B. thuringiensis grown on LB plate was used to inoculate a 3 ml of sterilised LB medium and incubated in a rotary shaker at 30 °C and 200 rates per minute overnight (14-18h). For inoculum preparation, 250 ml erlenmeyer flasks containing 50 ml of LB medium were inoculated with 1% (v/v) of the pre-inoculum and incubated in a rotary shaker at 30°C and 200 rates per minute for 6 h. The volume of culture inoculum was calculated on the basis of a final absorbance of 0.15 measured at 600 nm. The 250 ml shake flasks including 20 ml of complex low-cost medium were incubated with estimated inoculum volume. In such media, the initial OD was not measured after inoculation but calculated on the basis of the OD measured in the inoculum. Samples taken regularly from the incubated cultures were subjected to microscopical observation. The fermentation process was considered as ended when 90 % (or more) of the B. thuringiensis cells had been destroyed, liberating the crystals and spores.
2.4. Determination of delta-endotoxins
At the end of fermentation, one ml of collected samples was centrifuged at 13000×g for 10 min at a temperature of 4 °C. The supernatants were removed. The pellets were washed twice with 1 ml of 1 M NaCl solution and twice with 1 ml of bidistilled autoclaved water. The crystal proteins in the pellet were dissolved with 50mM NaOH (pH 12.5) for 2 h at 30 °C with vigorous shaking. The suspension was centrifuged at 13000×g for 10 min at 4 °C and the pellet was removed. The supernatant, containing the alkali-soluble insecticidal crystal proteins was used to define the delta-endotoxin concentration by Bradford method using bovine serum albumin as standard protein (Bradford 1976). Delta-endotoxins concentration was measured at 595 nm using a UV-visible spectrophotometer (Bio-Rad Laboratories, Inc.). The obtained values are the mean of triplicate runs of two separate experiments.
2.5. Statistical experimental design
The significance of the various media constituents towards delta-endotoxins production was further tested using Plackett-Burman statistical experimental designs (1946). This method is based upon the existence of Hadamard matrices, which are square matrices of order N with entries at two levels, +1 and −1. These matrices are orthogonal such that for each column the number of +1 is equal to the number of −1. This experimental design is suitable for screening the effect of a large number of factors in an experiment and ideal for the determination of main effects. The design assumes that there are no interactions between different media constituents, Xi, in the range of variables under consideration and a linear approach is considered sufficient for screening:
Where Y is the calculated target function and i are the regression coefficients.
With such experimental design, N factors can be screened with only N+1 trials and screening up to 100 variables (Castro et al. 1992) is feasible with the aid of this method. In the present study, effect of 7 factors of the medium on delta-endotoxin production was determined.
Trials were carried out in triplicate and at two different times and the responses considered for study represent mean of the responses. The effect of each variable was estimated by the standard equation:
Where R(H) characterizes all responses when constituent was at high levels, R(L) corresponds to all responses when constituent was in low levels, N is the total number of runs.
The standard error (S.E.) represents the square root of the variance of an effect and the (p-value) or significance level of each concentration effect is estimated using student’s t-test:
Where, E(xi) is the effect of the variable xi.
The coefficient of determination (R²) determines the degree of collinearity between simulated and measured data. Similarly, R² determines the proportion of the variance in experimental data explained by the model. R² ranges from 0 to 1, with higher values indicating less error variance, and typically values greater than 0.5 are considered acceptable (Santhi et al. 2001). Although R² have been widely used for model evaluation, these statistics are oversensitive to high extreme values (outliers) and insensitive to additive and proportional differences between model predictions and measured data (Legates and McCabe 1999).
3. Results and discussion
Table 1 represents the independent variables and their respective high and low concentrations used in the optimization study, whereas Table 2 represents the experimental design for 12 trials with two levels of concentrations for each variable, which was followed for the optimization of medium components for delta-endotoxins production.
Table 1: Medium components (variables) and the respective high and low concentration levels used in experimental design |
|||
Variable |
Medium components |
Lower level (g l-1) |
Higher level (g l-1) |
X1 |
KH2PO4 |
1.5 |
0.5 |
X2 |
K2HPO4 |
1.5 |
0.5 |
X3 |
MgSO4 |
0.1 |
0.5 |
X4 |
MnSO4 |
0 |
0.002 |
X5 |
FeSO4 |
0 |
0.002 |
X6 |
Starch |
25 |
35 |
X7 |
Soybean meal |
20 |
30 |
The variables X1, ..., X7 represented the medium constituents. Table 3 represents the results of statistical experiment with respect to endotoxins production, the effect, standard error, t and p-value (confidence level) of each component. The components were screened at the confidence level of 95% on the basis of their effects. If the component showed significance at or above 95% confidence level and its effect was negative, it indicated that the component was effective in delta-endotoxins production but the amount required was lower than the indicated as low (-) concentration in studied statistical experiment. If the effect was positive, a higher concentration than the indicated high value (+) concentration was required during further optimization studies. According to Table 2, delta-endotoxins concentrations ranged from 457 mg l-1 to 2426 mg l-1.
Table 2: Delta-endotoxins concentrations according to experimental design |
|||||||
KH2PO4 |
K2HPO4 |
MgSO4 |
MnSO4 |
FeSO4 |
starch |
soybean meal |
Delta-endotoxins (mg l-1) |
1,5 |
1,5 |
0,1 |
0,02 |
0 |
25 |
20 |
457±58 |
0,5 |
1,5 |
0,5 |
0,02 |
0 |
35 |
30 |
1808±64 |
0,5 |
0,5 |
0,5 |
0,02 |
0,02 |
25 |
30 |
1568±31 |
1,5 |
1,5 |
0,1 |
0,02 |
0,02 |
25 |
30 |
1671±55 |
1,5 |
0,5 |
0,5 |
0,02 |
0 |
35 |
20 |
915±47 |
0,5 |
0,5 |
0,1 |
0 |
0 |
25 |
20 |
1375±26 |
1,5 |
1,5 |
0,5 |
0 |
0,02 |
35 |
20 |
1368±28 |
1,5 |
0,5 |
0,5 |
0 |
0 |
25 |
30 |
1253±34 |
1,5 |
0,5 |
0,1 |
0 |
0,02 |
35 |
30 |
2426±57 |
0,5 |
1,5 |
0,5 |
0 |
0,02 |
25 |
20 |
1618±44 |
0,5 |
0,5 |
0,1 |
0,02 |
0,02 |
35 |
20 |
1626±20 |
0,5 |
1,5 |
0,1 |
0 |
0 |
35 |
30 |
601±36 |
The confidence level of all components were below 95% in delta-endotoxin production and hence, were considered insignificant (Table 3). Starch, considered as principal source of carbon, soybean meal (source of nitrogen), FeSO4 and MgSO4 enhanced the delta-endotoxin production whereas K2HPO4, KH2PO4 and MnSO4 inhibited the production of delta-endotoxins.
Table 3: Prediction model from regression analysis |
|||||
Predictor |
Coefficient |
SE |
t |
p |
Rank |
Constant |
208 |
1415 |
0.15 |
0,001 |
|
KH2PO4 |
-84.4 |
328.5 |
-0,26 |
0,810 |
6 |
K2HPO4 |
-273.2 |
328.5 |
-0,83 |
0,452 |
3 |
MgSO4 |
155.4 |
821.2 |
0,19 |
0,859 |
7 |
MnSO4 |
-4974 |
16424 |
-0,30 |
0,777 |
5 |
FeSO4 |
32248 |
16424 |
1.96 |
0,121 |
1 |
Starch |
13.37 |
32.85 |
0,41 |
0,705 |
4 |
Soybean meal |
32.77 |
32.85 |
1.00 |
0,375 |
2 |
S = 568.960 |
R² = 59.60% |
R²(adj) = 0,0%
|
|
|
|
Analysis of Variance (ANOVA) |
|
|
|
|
|
Source |
DF |
SS |
MS |
F |
p |
Regression |
7 |
1910312 |
272902 |
0,84 |
0,605 |
Residual Error |
4 |
1294861 |
323715 |
|
|
Total |
11 |
3205172
|
|
|
|
In fact, Nickerson and Bulla (1974) reported that the change in the carbon source affects the biological activity and the morphology of the crystals. The phenomenon could be possibly due to alteration on the rate of synthesis of the different Cry proteins (Yudina et al. 1992). On the other hand, free amino acids containing basic form of assimilated nitrogen by cell; have to be supplied in the medium in order to obtain good parasporal crystal formation in Bacillus thuringiensis (Egorov et al. 1984).
In addition, the regression is not linear (according to Table 3) because the amount of F-statistic for determination of effective factors on delta-endotoxin production is 0.84 with the significance level of p = 0.605. The results are presented in Table 3 which shows the regression model with coefficient of determination of 0.596 and adjusted coefficient of determination of 0 which means that constructed model explains two plausible cases: the first is that there is no linear competitive advantage between the seven components and the second is that the production is totally influenced by other factors.
The goodness of fit of a linear regression model is popularly measured by the sample coefficient of determination known as R². This measure has a tendency to rise and to reach its upper limit as the number of explanatory variables in the model increase. Such an uncomfortable feature is controlled to a large extent through an application of a correction for the degrees of freedom. This leads to another measure of the goodness of fitted model known as adjusted R². Both the high adjusted R² value and the close to zero p-value in the Analysis Of Variance (ANOVA) (Box et al. 1978; DeVor et al. 1992; Montgomery 2001) show that this model has a satisfactory goodness of fit. The use of R², the coefficient of determination, also called the multiple correlation coefficient, is well established in classical regression analysis (Rao 1973). Its definition as the proportion of variance explained by the regression model makes it useful as a measure of success of predicting the dependent variable from the independent variables. Generally, R² value is accepted as a standard criterion for the predictive success of the models (Hakanson et al. 2003). Multivariate models capable of assessing large number of variables and interrelations are therefore, more successful in defining and predicting biological processes such as delta-endotoxins production. In the present study, the relationships between nutrients having positive effect on delta-endotoxins production have been investigated using a linear multivariate approach. Thus, in order to increase secretion of delta-endotoxins by Bacillus thuringiensis strain, our major focus is to investigate the relationships between parameters having positive effect on delta-endotoxins production by Bacillus thuringiensis. In this study, MgSO4, FeSO4, starch and soybean meal will be more studied. The variable with confidence level above 95% is considered a significant factor. According to Table 4, we showed that all ingredients significantly affect the delta-endotoxin production independently for a significance level α=0.05.
Table 4: Prediction model (with interaction) from regression analysis
|
|||||
Predictor |
Coefficient |
SE |
t |
p |
Rank |
Constant |
4414.3 |
167.7 |
26.32 |
0.024 |
|
KH2PO4 |
-1061.24 |
38.27 |
-27.73 |
0,023 |
3 |
K2HPO4 |
703.69 |
38.27 |
18.39 |
0,035 |
8 |
MgSO4 |
4490.8 |
144.6 |
31.05 |
0,020 |
1 |
MnSO4 |
-29477 |
1446 |
-20.38 |
0,031 |
5 |
FeSO4 |
-367751 |
18398 |
-19.99 |
0,032 |
6 |
Starch |
-62.037 |
4.091 |
-15.16 |
0.042 |
10 |
Soybean meal |
-87.198 |
5.010 |
-17.40 |
0.037 |
9 |
FeSO4 × starch |
7302.4 |
375.8 |
19.43 |
0.033 |
7 |
FeSO4 × soybean |
10644.8 |
433.9 |
24.53 |
0.026 |
4 |
MgSO4 × FeSO4 |
-283982 |
9395 |
-30.23 |
0.021 |
2 |
S = 35.4291 |
R² =100.0% |
R²(adj) = 99,6% |
|
|
|
Analysis of Variance (ANOVA) |
|
|
|
|
|
Source |
DF |
SS |
MS |
F |
p |
Regression |
10 |
3203917 |
320392 |
255.25 |
0,049 |
Residual Error |
1 |
1255 |
1255 |
|
|
Total |
11 |
3205172 |
|
|
|
In order to obtain a higher R² value, we deduct from several constructed models that only three 2-factor interaction terms among these variables also significantly affect the delta-endotoxins production for α=0.05, i.e., (FeSO4 × MgSO4), (FeSO4 × starch), and (Soybean × FeSO4). The statistical significance of the second order equation was checked by an F-test (ANOVA) and data were shown in Table 4. The regression model for delta-endotoxins production was highly significant (p-value= 0.049) with a satisfactory value of determination coefficient (R2 = 1), indicating that total variability in the response could be explained. The closer the value of R2 is to 1.0, the stronger the model and the better it predicts the response. The model F-value of 255.25 indicated that the model terms were significant. Furthermore, the signs of the parameters in the model presented in Table 4 are examined. Positive signs mean that the production value will go in the same direction as the parameter, and negative signs imply the opposite. Therefore, the endotoxins production by Bacillus thuringiensis will be improved due to increases of MgSO4, K2HPO4, interaction of FeSO4 and soybean meal, and interaction of starch and FeSO4. Moreover, the positive effect of Magnesium ions on enhancement of delta-endotoxins production has been kept. In fact, the addition of macronutrients such as Mg2+ has been reported to increase the yield and insecticidal activities of B. thuringiensis (Goldberg et al 1980). However, interaction of FeSO4 and MgSO4 seems to decrease the production of delta-endotoxins. This finding suggests that when iron, coupled with magnesium ions, are able to prevent the formation of delta-endotoxins. Thus, on the basis of obtained results, we conclude that negative individual effects of starch, FeSO4, and soybean meal, were converted in positive effect when interactions were applied. Iron is an element required in trace amounts for most of the microorganisms in order to run their number of crucial biochemical reactions including reduction of the oxygen for synthesis of ATP, detoxification of oxygen radicals etc. These restrictions of availability under limited environmental conditions and biological requirements have disposed microorganisms to synthesize particular molecules that can compete with hydroxyl ion for the ferric state of iron, a nutrient which is present and biologically unavailable. Siderophores are low-molecular-mass (500-1000 Daltons) iron-chelating ligands that are produced by most microorganisms under iron-limited circumstances (Hider 1984), which bind ferric ions with high similarity (Neilands 1995). Siderophore solubilize the non-soluble surrounding iron in order to make it biologically available. Microorganisms, which produce siderophore have molecular systems to transport iron by siderophore – iron complexes into the cells through iron regulated outer membrane proteins (Braun et al. 1998). The obtained results may explain the positive relationship (FeSO4 × starch) and (FeSO4 × soybean) by the fact that Fe3+ agents have a highly specific chelating potency. Siderophores are synthesized by microorganisms to sequester iron from the culture medium, and carbon and nitrogen sources in a second time. The media used for the industrial production of B. thuringiensis are composed of complex carbon and nitrogen sources. Starch is considered as suitable carbon sources and a protein-rich material such as soybean flour is among the cheap sources of nitrogen used. Anderson (1990) suggested a relationship between carbon:nitrogen ratio and production of delta-endotoxins. According to Table 4, starch and soybean meal increased the delta-endotoxins production only when iron ions (Fe3+) were present in the culture medium. Several medium components such as nitrogen and carbon sources influence the metabolic and biochemical behaviour of the microbial strain and subsequent metabolite production pattern, such as delta-endotoxins secretion. Thus, the assimilation of Carbon and Nitrogen by Bacillus thuringiensis was more suitable with the presence of accessible iron ions. In planning a factorial experiment prior knowledge may suggest that some interactions are potentially important and should therefore be estimated free of the main effects.
4. Conclusion
In this study, the coefficient of determination (R²) has been considered as the most important criterion for better choice of interactions between culture components. Interaction permitted to discover hidden biological relationships between culture ingredients composing the bacterial environment and the studied microorganism. Our findings suggest that Iron is perhaps the most important micronutrient used by Bacillus thuringiensis strain and is essential for the metabolism. Iron actions have been highlight because of the potential role of microbial siderophores in facilitating uptake of iron ions and their mobilisation under specific growth conditions.
5. References
Anderson TB (1990) Effects of carbon: nitrogen ratio and oxygen on the growth kinetics of Bacillus thuringiensis and yield of bioinsecticidal crystal protein. Thesis, University of Western Ontario
Box GEP, Hunter WG, Hunter JS (1978) Statistics for Experimenters: Introduction to Design, Data Analysis, and Modelling Building, Wiley, New York.
Bradford M (1976) A rapid and sensitive method for the quantitation of microgram quantities of protein utilizing the principle of protein-dye binding. Anal Biochem 72:248-254
Braun V, Hantke K, Koster W (1998) Bacterial iron transport: mechanisms, genetics, and regulation. Met Ions Biol Syst 35:67–145
Bravo A, Gill SS, Soberon M (2007) Mode of action of Bacillus thuringiensis Cry and Cyt toxins and their potential for insect control. Toxicon 49: 423-435
Castro PML, Hayter PM, Ison AP, Bull AT (1992) Application of a statistical design to the optimization of culture medium for recombinant interferon-gamma production by Chinese hamster ovary cells. Appl Microbiol Biotechnol 38:84-90
DeVor RE, Chang T-H, Sutherland JW (1992) Statistical Quality Design and Control, Prentice Hall, Upper Saddle River
Dulmage HT, Rhodes RA (1982) Production of Bacteria in Artificial Media. In: Burges HD, Hussey H (eds) Microbial Control of Insects and Mites, Academic Press, London, New York, pp 507-540
Egorov NS, Al-Nuri MA, Prianishnikova NI (1984) Selection of Streptomyces spheroides-a producer of extracellular proteases with fibrinolytic action. Mikrobiologiia 53: 387-391
Ennouri K, Ben Khedher S, Jaoua S, Zouari N (2013) Correlation between delta-endotoxin and proteolytic activities produced by Bacillus thuringiensis var. kurstaki growing in an economic production medium. Biocontrol Sci Techn 23:756-767
Ghribi D, Zouari N, Trigui W, Jaoua S (2007) Use of sea water as salts source in starch and soya bean based media for production of Bacillus thuringiensis bioinsecticides. Process Biochem 42:374-378
Goldberg I, Sneh B, Battae E, Klein D (1980) Optimization of a medium for a high production of spore-crystal preparation of Bacillus thuringiensis effective against egyptian cotton leafworm Spodoptera /ittorallis. Biotech Lett 2: 419-426
Hakanson L, Malmaeus JM, Bodemer U, Gerhardt V (2003) Coefficients of variation for chlorophyll, green algae, diatoms, cryptophytes and blue-greens in rivers as a basis for predictive modelling and aquatic management. Ecol Modell 169: 179-196
Hider RC (1984) Siderophore mediated absorption of iron. Struct Bond 58:26-87
Holmberg A, Sievanen R, Carlberg G (1980) Fermentation of Bacillus thuringiensis for exotoxin production: Process Analysis study. Biotechnol Bioeng 22:1707-1724
Legates DR, McCabe GJ (1999) Evaluating the use of “goodness-of-fit” measures in hydrologic and hydroclimatic model validation. Water Resources Res 35: 233-241
Montgomery DC (2001) Design and Analysis of Experiments. Wiley, New York
Neilands JB (1995) Siderophores: structure and function of microbial iron transport compounds. J Biol Chem 270:26723–26726
Nickerson KW, Bulla LA (1974) Physiology of spore-forming bacteria associated with insects: minimal nutrition requirements for growth, sporulation and parasporal crystal formation of Bacillus thuringiensis. Appl Microbiol 28:124-128
Plackett RL, Burman JP (1946) The design of optimum multifactorial experiments. Biometrika 33:305-325
Rao CR (1973) Linear Statistical Inference and its Applications. Wiley, New York
Santhi C, Arnold JG, Williams JR, Dugas WA, Srinivasan R, Hauck LM (2001) Validation of the SWAT model on a large river basin with point and nonpoint sources. J American Water Resources Assoc 37:1169-1188
Schnepf E, Crickmore N, Van Rie J, Lereclus D, Baum J, Feitelson J, Zeigler DR, Dean DH (1998) Bacillus thuringiensis and its pesticidal crystal proteins. Microbiol Mol Biol Rev 62: 775-806
Yudina TG, Salamakha OV, Olekhnovich EV, Rogstykh NP, Egorov NS (1992) Effect of carbon source on the biological activity and morphology of paraspore crystals from Bacillus thuringiensis. Microbiology 61:402-407